Translate this page into:
Identification of differentially expressed genes and screening for key genes involved in ovarian cancer prognosis: An integrated bioinformatics and network analysis approach
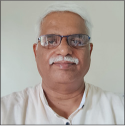
*Corresponding author: Prof. Samir Kumar Patra, Department of Life Science, National Institute of Technology, Rourkela, Odisha, India. samirp@nitrkl.ac.in
-
Received: ,
Accepted: ,
How to cite this article: Niharika, Roy A, Patra SK. Identification of differentially expressed genes and screening for key genes involved in ovarian cancer prognosis: An integrated bioinformatics and network analysis approach. J Reprod Healthc Med. 2024;5:8. doi: 10.25259/JRHM_6_2024
Abstract
Objectives:
Ovaries are important and essential organs of animals in producing and releasing eggs. Ovarian cancer (OvCa) is one of the most prevalent lethal gynecological malignancies with a lack of distinct biomarkers. Advances in high-throughput genomic data and the continued refinement of bioinformatics tools enable the identification of potential biomarkers. Leveraging these insights, we can employ systems biology approaches to enhance the accuracy of diagnosis and prognosis.
Material and Methods:
A comparative analysis was conducted between normal and tumor samples, employing bioinformatics software and tools. Differential expression analysis utilized fold-change statistics, while DAVID 6.8 software was used to perform gene ontology enrichment analysis and Kyoto Encyclopedia of Genes and Genomes pathway enrichment analyses. The protein-protein interaction (PPI) network was constructed differentially expressed genes (DEGs) using Search Tool for the Retrieval of Interacting Genes database, and Cytoscape 3.9.1, along with its Molecular Complex Detection and CytoHubba plugins, facilitated network visualization, analysis, and module detection. Hub gene expression and overall survival were explored through the Kaplan–Meier plotter, while Gene Expression Profiling Interactive Analysis 2 analyzed the tumor stage of OvCa patients. Hub genes protein expression was analyzed using the human protein atlas database through immunostaining results. The NetworkAnalyst program and Cytoscape were employed to analyze and visualize the transcription factor-hub gene associations. Subsequently, single-nucleotide variation, methylation, and pathway activity of hub genes were examined. Validation of hub genes messenger RNA expression was done using quantitative real-time polymerase chain reaction analysis.
Results:
607 DEGs, including 248 upregulated and 359 downregulated genes, were identified. The top 20 candidate genes were screened out through PPI network analysis. We discovered that the genes BUB1 Mitotic Checkpoint Serine/Threonine Kinase B (BUB1B), Cyclin A2 (CCNA2), Mitotic Arrest Deficient 2 Like 1 (MAD2L1), Protein Regulator of Cytokinesis 1 (PRC1), Thyroid Hormone Receptor Interactor 13 (TRIP13), and ZW10 Interacting Kinetochore Protein (ZWINT) exhibited significant importance in OvCa prognosis.
Conclusion:
Six genes, BUB1B, CCNA2, MAD2L1, PRC1, TRIP13, and ZWINT (identified as functional hub genes), are probably playing tumor-promotive roles, except TRIP13. All genes product is functionally related to the cell cycle. These can be targeted in quest of potential therapeutics for OvCa treatment.
Keywords
Bioinformatic analysis
Ovarian cancer
BUB1 mitotic checkpoint serine/threonine kinase B
Cyclin A2
Mitotic arrest deficient 2 like 1
Protein Regulator of Cytokinesis 1
Thyroid hormone receptor interactor 13
ZW10 interacting kinetochore protein
INTRODUCTION
Ovarian cancer (OvCa) is the most common and fatal cancer among women worldwide, ranking fifth in cancer-related deaths.[1,2] Unfortunately, most patients are diagnosed at advanced stages. According to the International Agency for Research on Cancer, the number of new OvCa cases globally is projected to rise from 324,603 to 476,893 between 2022 and 2050, with mortality estimates ranging from 206,956 to 328,287.[3] OvCa originates from three primary cell layers: surface epithelium, germ cells, and stromal cells, with epithelial OvCa being the most prevalent, constituting 85–90% of all cases. There are over 30 different types of OvCa based on their cell origins, which can be misinterpreted if not carefully traced. The primary subtypes of epithelial OvCa include high-grade serous ovarian cancer, low-grade serous ovarian cancer, clear cell tumors, mucinous tumors, and endometrioid tumors. Notably, 50% of new cases occur in women aged 65 and older (https://ocrahope.org/get-the-facts/statistics).[4-6]
OvCa poses a significant health challenge for women worldwide due to its complex origins, distinctive metastatic pattern, and varying disease spectrums. Family history, age, and germline mutations in BRCA1 and BRCA2 genes significantly contribute to the risk, accounting for 10–20% of cases.[7] Primary factors contributing to OvCa progression include age, family history, infertility, obesity, in vitro fertilization, hormonal replacement therapy, sedentary lifestyle, and endometriosis, which can be classified as genetic or environmental influences.[8] In 2023, the SEER program recorded 19,710 new OvCa cases (1.0% of all cancers) and 13,270 deaths (2.2% of cancer-related mortalities), with a 5-year survival rate of 50.9% from 2014 to 2020 [https://seer.cancer.gov/statfacts/html/ovary.html, Figure 1]. Survival rates are significantly influenced by the stage of diagnosis, with early-stage detection leading to better treatment outcomes and higher survival rates than advanced stages; 18.2% of cases diagnosed at a localized stage have a five-year relative survival rate of 92.4% [Figure 2].
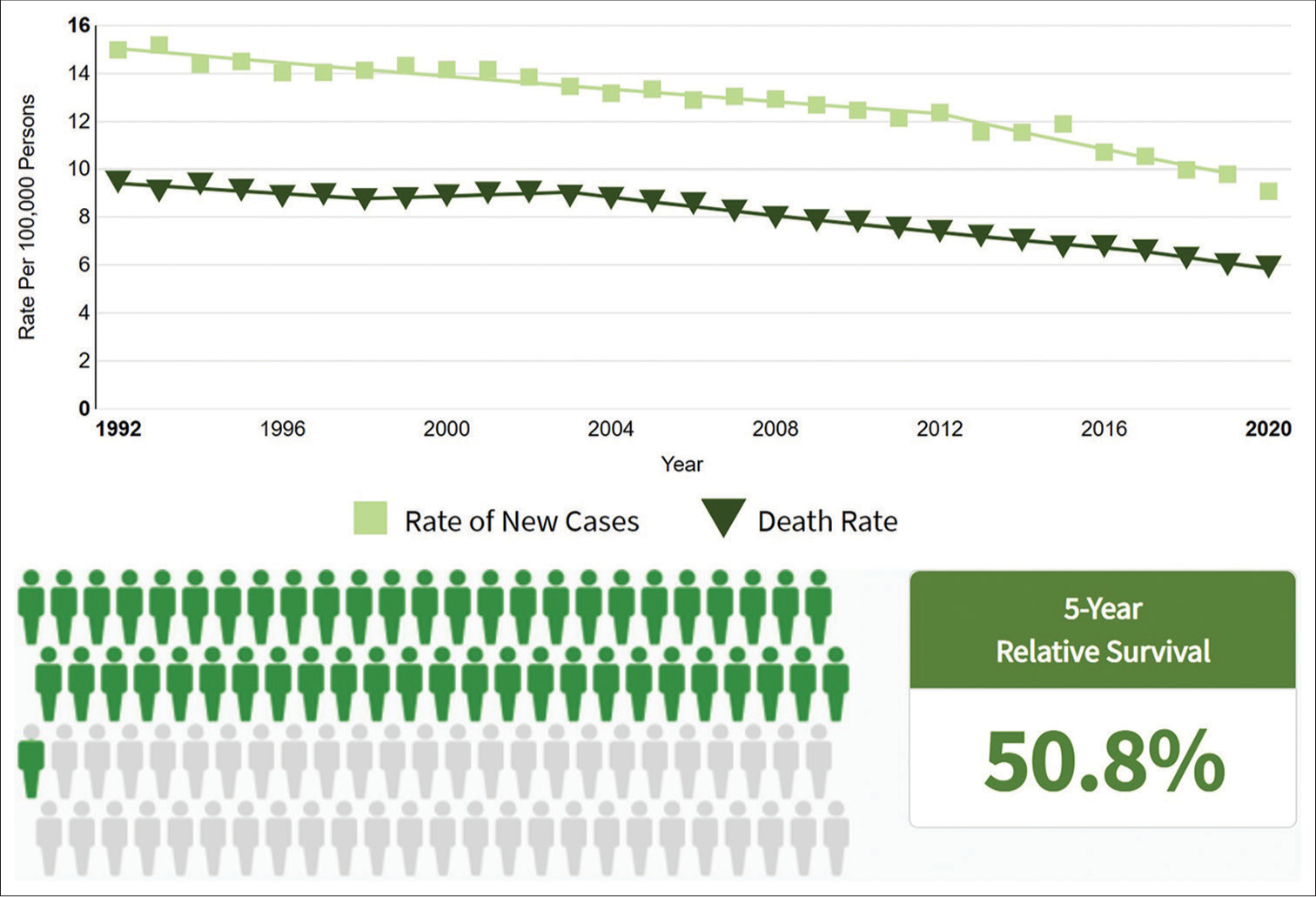
- Estimated ovarian cancer cases reported in 2022 with a 5-year survival percentage.
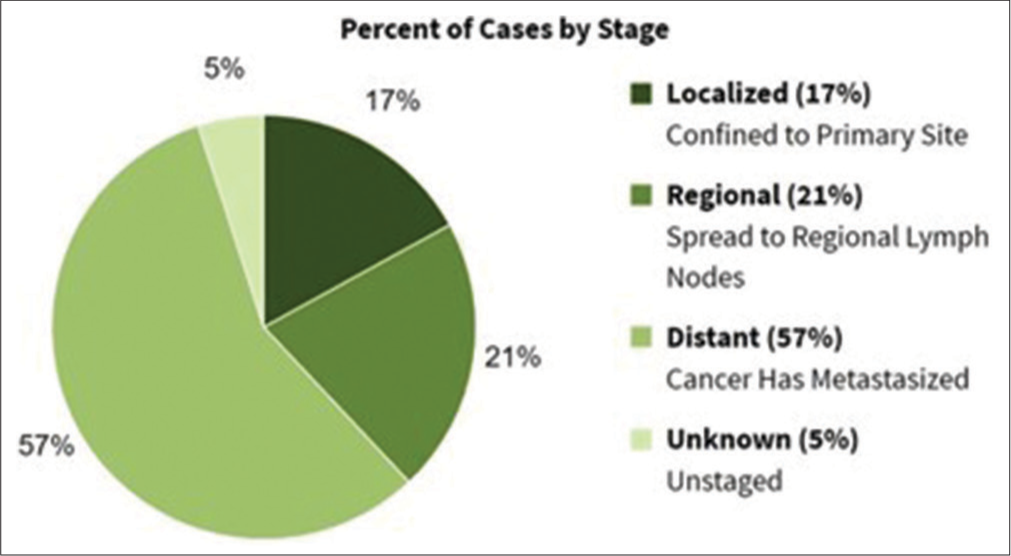
- Relative survival by stages at diagnosis in ovarian cancer.
OvCa screening employs several diagnostic methods that are categorized into three main areas. First, cancer antigen-125 (CA-125), a high molecular weight glycoprotein, monitors epithelial OvCas, with levels rising significantly as the tumor grows.[9] Second, imaging techniques such as transvaginal sonography, Doppler ultrasonography, computerized tomography scans, and ultrasounds are used for detailed analysis. Third, combined tests such as the risk of malignancy index and the risk of malignancy algorithm, which incorporate CA-125 and human epididymis-4 levels, provide enhanced screening accuracy. In addition, the Food and Drug Administration-approved serum OVA-1 test measures CA-125, transferrin, b2-microglobulin, prealbumin, and apolipoprotein A1 levels to assess the complexity of malignancy.[10-14]
Microarray technology and bioinformatics play pivotal roles in unraveling genetic alterations underlying tumor development and cancer progression. These tools aid in identifying new diagnostic and prognostic biomarkers for various cancer types. Public database platforms such as the National Center for Biotechnology Information (NCBI)-Gene Expression Omnibus (GEO) facilitate bioinformatics analysis, enabling the study of molecular mechanisms driving ovarian carcinoma.[15,16] To delve deeper into this area and gain fresh perspectives, we acquired three datasets, namely, GSE18521, GSE26712, and GSE54388, from the publicly accessible GEO database. Using the GEO2R tool, we identified DEGs between OvCa samples and normal ovarian tissues across these datasets. Subsequently, employing Venn diagram software, we pinpointed the common upregulated and downregulated genes [Figure 3].[17,18] Following this initial analysis, we proceeded to perform functional enrichment analysis and constructed a protein-protein interaction (PPI) network. To validate our findings, we utilized various online tools including the Kaplan–Meier plotter (KM-plotter), human protein atlas (HPA) database, Gene Expression Profiling Interactive Analysis 2 (GEPIA2, http://gepia2.cancer-pku.cn/), the University of Alabama at Birmingham CANcer (UALCAN, http://ualcan.path.uab.edu/index.html) data analysis portal, and Gene Set Control Analysis (GSCA, [http://bioinfo.life.hust.edu.cn/GSCA/#/]) web tools. These tools helped confirm the significance of selected hub genes and facilitated the construction of a transcription factor (TF)-gene regulatory network (GRN). In summary, this bioinformatics-driven study aims to identify potential biomarkers for the early detection of OvCa patients.
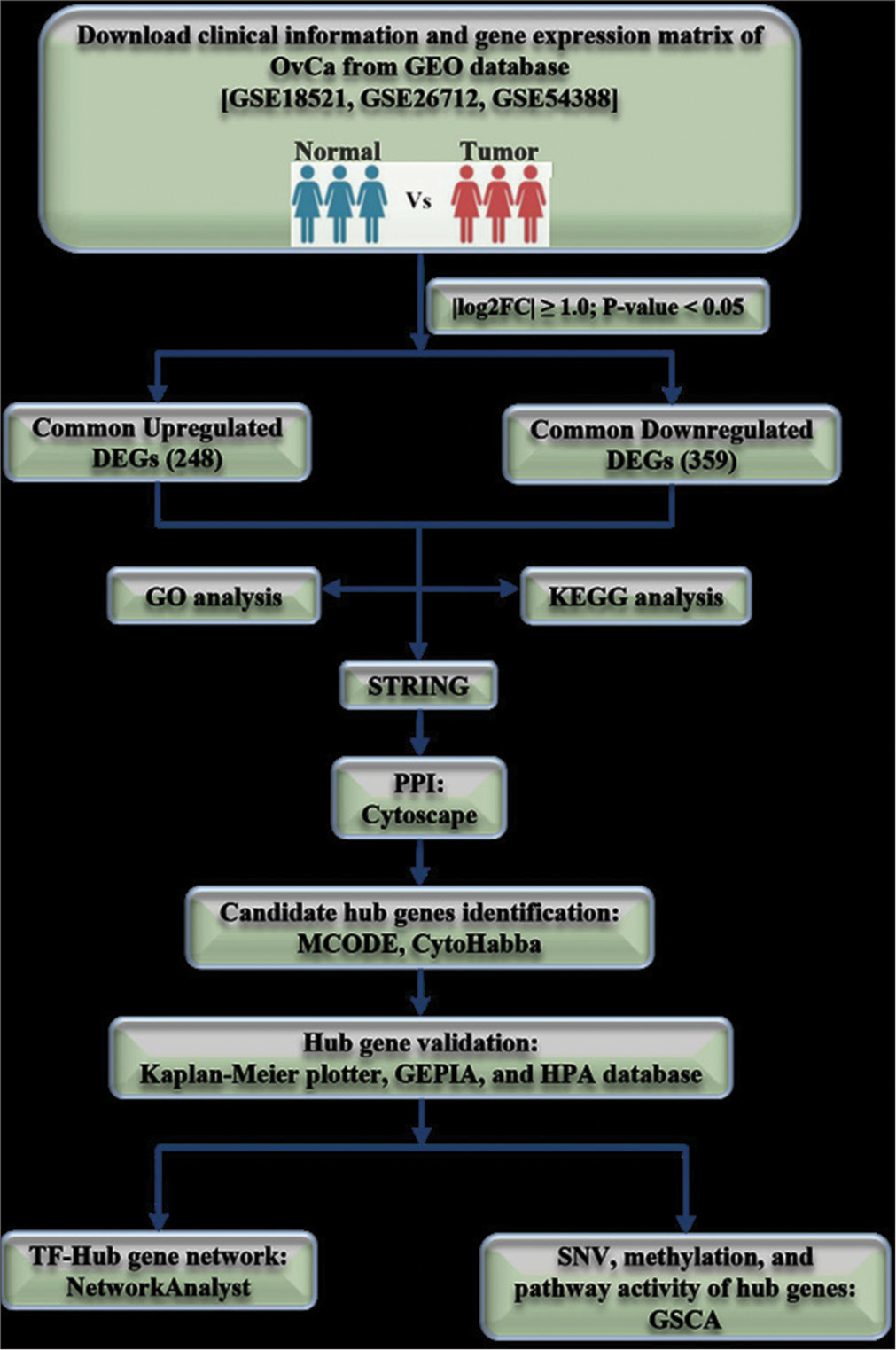
- Flow chart of the study.
MATERIAL AND METHODS
Microarray data information
In the GEO database, “Epithelial ovarian cancer” was searched and selected, “Homo sapiens” in the “Top Organisms,” in the “Study type,” “Expression profiling by array” was selected. The inclusion articles should meet the following criteria: (I) Each dataset included an OvCa sample group and control or healthy sample group, and (II) minimum two samples were included in each group. Exclusion criteria were as follows: (I) Duplicate studies, (II) letters, (III) review articles, (IV) case reports, (V) incomplete data, (VI) non-human studies, and (VII) datasets lacking control sample group. Three microarray expression profile datasets, GSE18521,[19] GSE26712,[20,21] and GSE54388,[22] were downloaded [details of datasets at Table 1].
Identification of DEGs
Differential expression analysis between ovarian carcinoma and normal ovarian samples was conducted using the GEO2R web-based tool provided by NCBI-GEO (http://www.ncbi.nlm.nih.gov/geo/geo2r). Key regulators with distinct expression patterns were identified based on fold change (FC) statistics and P-value measures. A P-value threshold of <0.05 was applied, and FC, a widely used method for differential expression analysis, was calculated to quantify the magnitude of gene expression changes between OvCa and control samples in a case-control analysis. FC represents the ratio of expression averages between control and disease samples and is expressed as a logarithm (logFC). Typically, a logFC ≥ −1.0 indicates down-regulation, while a logFC ≥ +1.0 suggests upregulation. A list of candidate genes identified as DEGs was subjected to further downstream analysis. Subsequently, 607 DEGs were found to be common across all three datasets, as determined using Venn diagram online software (https://bioinfogp.cnb.csic.es/tools/venny/)
Enrichment analyses of DEGs
The DEGs identified across the three GEO datasets were subjected to additional analysis for Gene Ontology (GO) and Kyoto Encyclopedia of Genes and Genomes (KEGG) pathway enrichment. A significance threshold of P < 0.05 was applied. GO enrichment analysis categorizes genes into predefined functional classes based on their biological characteristics. Tools such as DAVID,[23] PANTHER,[24] and GeneWeaver[25] are commonly used for GO enrichment analysis. For this study, we utilized the latest version of DAVID (6.8).
PPI network and significant module analysis
Creating GRNs is crucial for conducting further topological analysis, identifying modules, and pinpointing highly influential genes (hub genes), which could serve as potential targets for cancer prognosis. To reconstruct the GRN, we employed the Search Tool for the Retrieval of Interacting Genes (STRING) database (https://string-db.org/) (Version 11.5), a publicly accessible resource.[26] The STRING database serves as a comprehensive repository for exploring and analyzing functional connections between proteins, often inferred from genomic associations among the encoding genes. At present, it contains 67,592,464 proteins from 14,094 organisms, with over 20 billion interactions. The database predicts functional interactions with an expected accuracy level of at least 80% for more than half of the genes. We uploaded the list of DEGs to the STRING and utilized default parameter settings to retrieve interactions.
On retrieving the interaction network from STRING, we utilized the Cytoscape 3.9.1 software tool for network visualization and topological analysis.[27] The NetworkAnalyzer plugin of Cytoscape was employed to compute various topological properties of the constructed network.[28]
Network module identification
Identifying functional units within complex biological networks, such as network modules or biological pathways, is a fundamental challenge. Due to the immense size and complexity of biological networks, module identification is crucial for gaining meaningful biological insights. Module identification, also known as community detection or graph clustering, involves various software tools and packages designed for this purpose.
In our study, we utilized the Molecular Complex Detection (MCODE) algorithm, a widely used clustering algorithm, along with the CytoHubba plugin. CytoHubba offers 11 topological analysis methods, including Degree, Edge Percolated Component, Maximum Neighborhood Component, Density of Maximum Neighborhood Component, Maximal Clique Centrality, and six centralities (Bottleneck, EcCentricity, Closeness, Radiality, Betweenness, and Stress) based on shortest paths. Among these methods, the newly proposed maximal clique centrality (MCC) method has shown superior performance in predicting essential proteins within biological networks. Through MCODE, we identified highly interconnected regions within the network, often representing protein complexes or segments of pathways. MCODE employs a vertex-weighting approach based on the clustering coefficient (Ci = 2n/ki[ki=1]), where “ki” denotes the vertex size of the neighborhood of vertex “I” and “n” represents the number of edges in the neighborhood.[29,30]
Validation of candidate hub genes
To assess the impact of identified OvCa gene biomarkers/hub genes on patient survival, we utilized the KM plotter (http://kmplot.com/analysis) to generate survival plots. The KM plotter is a global online platform that mines microarray data from sources such as the GEO and the Cancer Genome Atlas (TCGA) to evaluate the prognostic power of genes in OvCa patients. The TCGA project offers a wealth of samples across various cancer types, enabling comprehensive molecular characterization and exploration of tumor heterogeneity.
To validate differences in hub gene expression patterns between normal and cancerous tissues, we employed the GEPIA2 database[31], which draws on thousands of samples from TCGA and the Genotype-Tissue Expression projects.[32] In addition, we utilized the HPA database (https://www.proteinatlas.org/) to compare protein expression levels of hub genes between cancer specimens and normal specimens. The HPA database comprises over 10 million images showcasing human protein expression patterns in tissues and cells, providing researchers with a freely accessible resource for studying the human proteome.[33]
TF-GRN construction
NetworkAnalyst (https://www.networkanalyst.ca) serves as a robust, all-encompassing web-based platform designed for statistical analysis, visualization, network analysis, and meta-analysis of gene expression data.[34,35] We utilized this tool to acquire information regarding the direct interactions between TFs and hub genes, which was then downloaded and visualized using Cytoscape.
Relationship between hub genes expression and their effect on pathway activity
GSCA is a web-based platform dedicated to genomic cancer analysis, focusing on gene set cancer analysis, dynamic analysis, visualization of gene sets related to malignant tumor gene expression, and correlation with drug sensitivity.
Cell culture
Two cell lines were taken for the validation of our study. One is human epidermal keratinocytes (HaCaT), immortalized human keratinocytes as control, and another is a human OvCa cell line, SK-OV-3 (SKOV3) (kindly given by Prof. Sib Sankar Roy, CSIR-IICB). SKOV3 and HaCaT cells were maintained in RPMI 1640 (Gibco, Cat # 31800022) and DMEM (Gibco, Cat # 12100046), respectively, supplemented with 10% fetal bovine serum (Gibco, Cat # 10270106) and penicillin (100 unit/mL) streptomycin (0.1 mg/mL) (HiMedia, SKU: A002A).
RNA extraction and quantitative real-time polymerase chain reaction (qRT-PCR) analysis
Total cellular RNA was isolated from each cell line using the TRIzol method (Thermo Scientific) as per the manufacturer’s protocol. Subsequently, cDNA was synthesized from 1 μg of total RNA using the GeneSure First Strand cDNA Synthesis Kit (Pure-gene, Genetix). qRT-PCR was performed using PowerUp™ SYBR™ Green Master Mix in the Agilent Technologies MX300SP RT-PCR system. The messenger RNA (mRNA) expression levels were normalized to the housekeeping gene b-Actin, which served as an endogenous control. Calculations were conducted using the 2−ΔΔCt method.
Statistical analysis
The data for this study were obtained following the recommended statistical approach outlined in the respective databases. Gene expression datasets were acquired from the GEO database and analyzed utilizing GEO2R. Statistical analysis was conducted using an unpaired sample t-test. The findings were presented as mean ± standard deviation, with statistical significance denoted by P < 0.05.
RESULTS
Identification of DEGs in OvCa
Differential expression analysis identified a total of 3342 DEGs in GSE18521, with 1846 upregulated and 1496 downregulated genes. In GSE26712, 1000 DEGs were identified, comprising 318 upregulated and 682 downregulated genes. In addition, 1045 DEGs were detected in GSE54388, with 821 upregulated and 224 downregulated genes. Subsequently, utilizing Venn diagram software, we identified 248 commonly upregulated and 359 commonly downregulated DEGs across all three datasets [Figure 4], indicating consistent expression patterns in OvCa samples compared to non-cancerous samples.
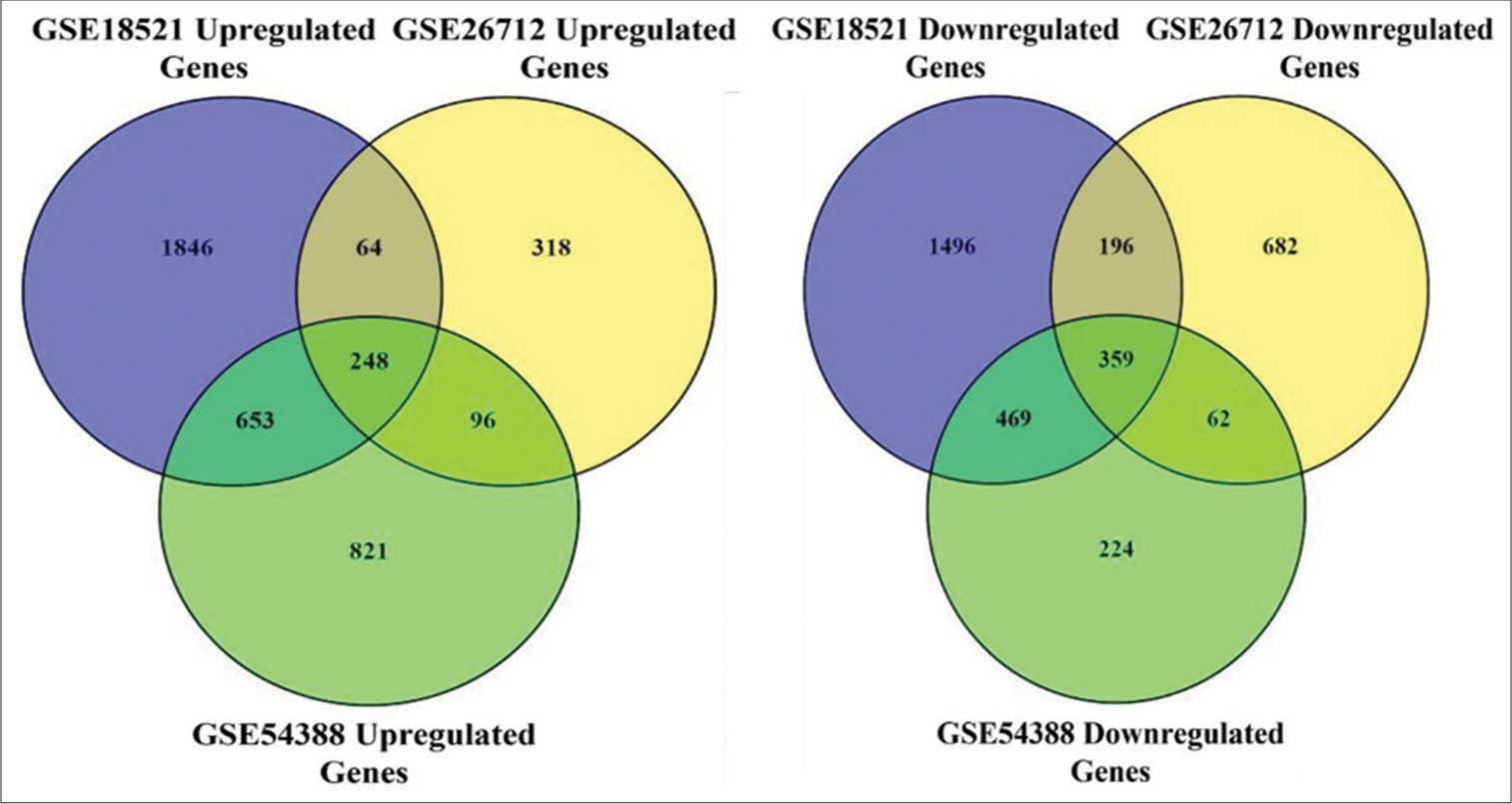
- Identification of differentially expressed genes in three gene expression omnibus (GEO) datasets. Left Venn diagram of 248 overlapping upregulated genes from the intersection of three independent GEO datasets. P < 0.05 and |log FC |>1 was set as the threshold. Right Venn diagram of 359 overlapping downregulated genes from the intersection of three independent GEO datasets. P < 0.05 and |log FC |>1 was set as the threshold.
KEGG and GO enrichment analyses
Functional pathway enrichment analyses of DEGs were performed using DAVID. The GO analysis [Table 2] revealed significant (P < 0.05) enrichments in various biological processes (BPs), including response to drug (GO:0042493), cell division (GO:0051301), cell adhesion (GO:0007155), and mitotic spindle assembly checkpoint (GO:0007094). Molecular function analyses highlighted enrichments in protein binding (GO:0005515), identical protein binding (GO:0042802), protein homodimerization activity (GO:0042803), and heparin binding (GO:0008201). Cell component (CC) analyses identified enrichments in extracellular space (GO:0005615), cytosol (GO:0005829), extracellular exosome (GO:0070062), and extracellular region (GO:0005576).
Category | Term | Count | % | P-value | FDR |
---|---|---|---|---|---|
GO analysis | |||||
GOTERM_BP_DIRECT | GO: 0042493~response to drug | 30 | 5.102040816 | 1.46E-08 | 5.25E-05 |
GOTERM_BP_DIRECT | GO: 0051301~cell division | 32 | 5.442176871 | 4.31E-07 | 7.74E-04 |
GOTERM_BP_DIRECT | GO: 0007155~cell adhesion | 40 | 6.802721088 | 6.61E-07 | 7.91E-04 |
GOTERM_BP_DIRECT | GO: 0007094~mitotic spindle assembly checkpoint | 9 | 1.530612245 | 3.15E-06 | 0.002143 |
GOTERM_BP_DIRECT | GO: 0071560~cellular response to transforming growth factor beta stimulus | 12 | 2.040816327 | 3.41E-06 | 0.002143 |
GOTERM_BP_DIRECT | GO: 0045944~positive regulation of transcription from RNA polymerase II promoter | 65 | 11.05442177 | 3.58E-06 | 0.002143 |
GOTERM_BP_DIRECT | GO: 0006915~apoptotic process | 40 | 6.802721088 | 5.27E-06 | 0.002703 |
GOTERM_BP_DIRECT | GO: 0008285~negative regulation of cell proliferation | 32 | 5.442176871 | 1.89E-05 | 0.008497 |
GOTERM_BP_DIRECT | GO: 0000079~regulation of cyclin-dependent protein serine/threonine kinase activity | 10 | 1.700680272 | 2.27E-05 | 0.00906 |
GOTERM_BP_DIRECT | GO: 0009410~response to xenobiotic stimulus | 21 | 3.571428571 | 3.50E-05 | 0.012568 |
GOTERM_CC_DIRECT | GO: 0005615~extracellular space | 113 | 19.21768707 | 1.22E-13 | 6.48E-11 |
GOTERM_CC_DIRECT | GO: 0005829~cytosol | 233 | 39.62585034 | 1.20E-12 | 3.17E-10 |
GOTERM_CC_DIRECT | GO: 0070062~extracellular exosome | 120 | 20.40816327 | 3.08E-12 | 5.45E-10 |
GOTERM_CC_DIRECT | GO: 0005576~extracellular region | 111 | 18.87755102 | 2.30E-10 | 3.04E-08 |
GOTERM_CC_DIRECT | GO: 0005737~cytoplasm | 217 | 36.9047619 | 4.92E-08 | 5.22E-06 |
GOTERM_CC_DIRECT | GO: 0005886~plasma membrane | 199 | 33.84353741 | 1.50E-07 | 1.33E-05 |
GOTERM_CC_DIRECT | GO: 0016020~membrane | 115 | 19.55782313 | 3.32E-07 | 2.52E-05 |
GOTERM_CC_DIRECT | GO: 0016328~lateral plasma membrane | 13 | 2.210884354 | 8.92E-07 | 5.91E-05 |
GOTERM_CC_DIRECT | GO: 0005634~nucleus | 217 | 36.9047619 | 7.02E-06 | 3.88E-04 |
GOTERM_CC_DIRECT | GO: 0005654~nucleoplasm | 155 | 26.36054422 | 7.33E-06 | 3.88E-04 |
GOTERM_MF_DIRECT | GO: 0005515~protein binding | 449 | 76.36054422 | 7.47E-12 | 6.41E-09 |
GOTERM_MF_DIRECT | GO: 0042802~identical protein binding | 98 | 16.66666667 | 5.38E-10 | 2.31E-07 |
GOTERM_MF_DIRECT | GO: 0042803~protein homodimerization activity | 46 | 7.823129252 | 2.18E-06 | 6.24E-04 |
GOTERM_MF_DIRECT | GO: 0008201~heparin binding | 19 | 3.231292517 | 6.05E-06 | 0.001297 |
GOTERM_MF_DIRECT | GO: 0019901~protein kinase binding | 34 | 5.782312925 | 3.16E-05 | 0.004535 |
GOTERM_MF_DIRECT | GO: 0045296~cadherin binding | 25 | 4.25170068 | 3.17E-05 | 0.004535 |
GOTERM_MF_DIRECT | GO: 0005509~calcium ion binding | 43 | 7.31292517 | 8.55E-05 | 0.010477 |
GOTERM_MF_DIRECT | GO: 0016491~oxidoreductase activity | 20 | 3.401360544 | 1.28E-04 | 0.012835 |
GOTERM_MF_DIRECT | GO: 0019899~enzyme binding | 27 | 4.591836735 | 1.35E-04 | 0.012835 |
GOTERM_MF_DIRECT | GO: 0004866~endopeptidase inhibitor activity | 8 | 1.360544218 | 3.54E-04 | 0.02802 |
KEGG_PATHWAY | |||||
KEGG_PATHWAY | hsa05200:Pathways in cancer | 49 | 8.333333 | 1.43E-08 | 4.12E-06 |
KEGG_PATHWAY | hsa04110:Cell cycle | 20 | 3.401361 | 2.63E-07 | 3.78E-06 |
KEGG_PATHWAY | hsa04510:Focal adhesion | 22 | 3.741497 | 2.60E-05 | 0.002495 |
KEGG_PATHWAY | hsa05205:Proteoglycans in cancer | 22 | 3.741497 | 3.50E-05 | 0.002517 |
KEGG_PATHWAY | hsa04390:Hippo signaling pathway | 16 | 2.721088 | 9.59E-04 | 0.037954 |
KEGG_PATHWAY | hsa00830:Retinol metabolism | 10 | 1.70068 | 0.001054277 | 0.037954 |
KEGG_PATHWAY | hsa04114:Oocyte meiosis | 13 | 2.210884 | 0.004280404 | 0.087529 |
KEGG_PATHWAY | hsa04151:PI3K-Akt signaling pathway | 25 | 4.251701 | 0.004507603 | 0.087529 |
KEGG_PATHWAY | hsa04010:MAPK signaling pathway | 19 | 3.231293 | 0.032692855 | 0.254849 |
KEGG_PATHWAY | hsa04210:Apoptosis | 11 | 1.870748 | 0.035395711 | 0.254849 |
The results are sorted and arranged in the ascending order of P<0.05. GO: Gene ontology, KEGO: Kyoto encyclopedia of genes and genomes, FDR: False discovery rate
Furthermore, KEGG pathway enrichment analysis revealed significant enrichments in pathways such as hsa05200 (pathways in cancer), hsa04110 (Cell cycle), hsa04510 (Focal adhesion), and hsa05205 (Proteoglycans in cancer). These findings were corroborated by the PPI network and significant module analysis.
Reconstruction and analysis of cancer network
To reconstruct the regulatory network, we employed the STRING database, yielding a total of 599 nodes and 3286 edges. The average node degree was 11, while the average local clustering coefficient stood at 0.352. Notably, the PPI enrichment P-value was found to be < 1.0e-16 [Figure 5]. Subsequently, Cytoscape software was used for network visualization and topological analysis. Utilizing the NetworkAnalyzer plugin, we computed various topological properties of the reconstructed network, as summarized in Table 3.
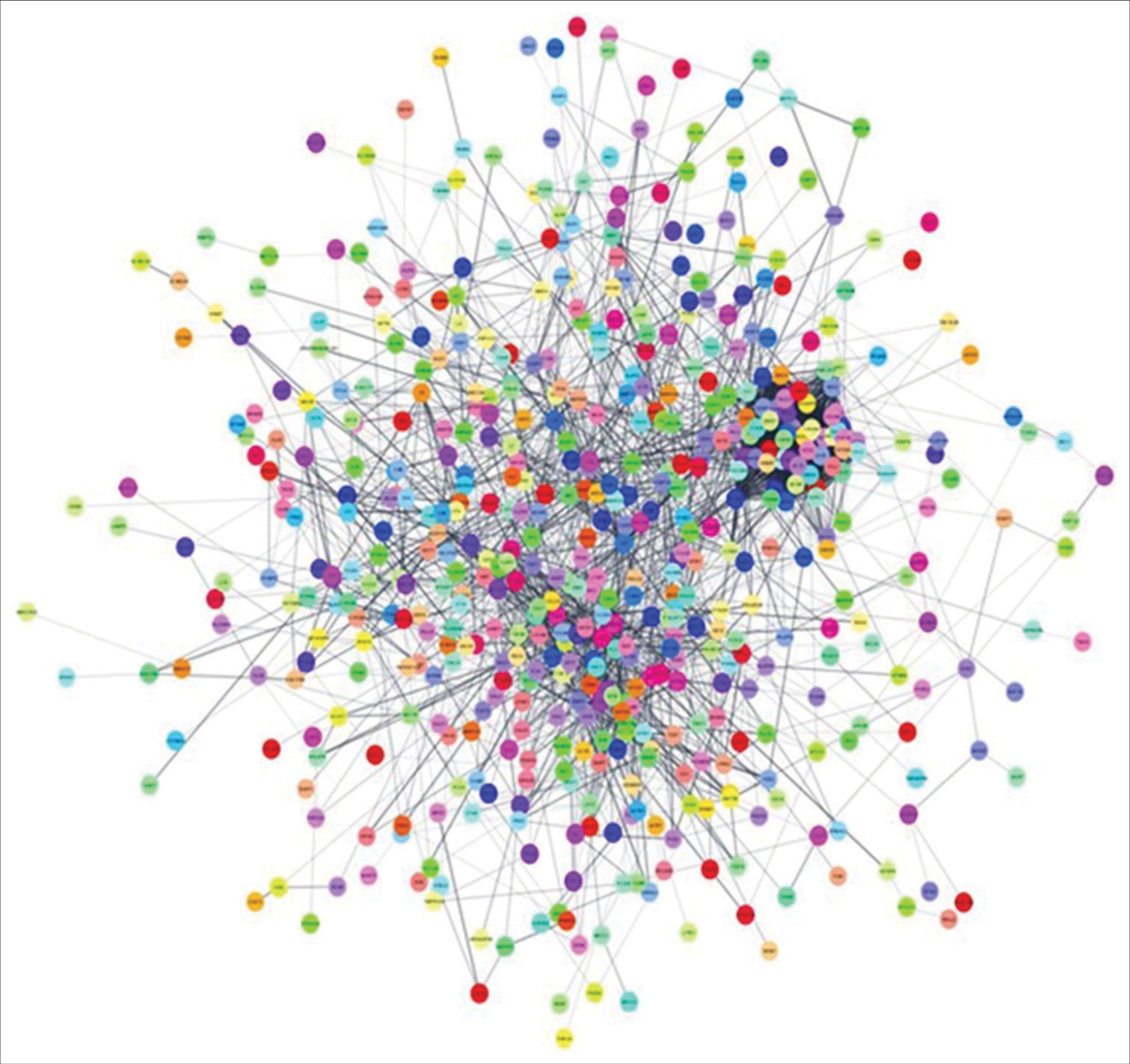
- PPI network: PPI network of overlapping differentially expressed genes was constructed in the STRING database and visualized in Cytoscape. PPI: Protein-protein interactions, STRING: Search tool for the retrieval of interacting genes.
Topological parameters | Value |
---|---|
Number of nodes | 599 |
Number of edges | 3286 |
Avg. number of neighbors | 11,792 |
Network diameter | 9 |
Network radius | 5 |
Characteristic path length | 3521 |
Clustering coefficient | 0.278 |
Network density | 0.021 |
Network heterogeneity | 1.271 |
Network centralization | 0.109 |
Connected components | 41 |
Module identification in the OvCa network
The MCODE network clustering algorithm was applied to the reconstructed OvCa network with default parameters (degree cutoff = 2, node score cutoff = 0.2, haircut = true, fluff = false, k-core = 2, and max. depth = 100), resulting in the identification of fourteen clusters. Further, analysis focused on the top four modules [Figure 6a]. Subsequently, the MCC method of CytoHubba was utilized to select the top 20 genes, which included Baculoviral Inhibitor of Apoptosis Repeat-Containing 5 (BIRC5), BUB1, Budding Uninhibited by Benzimidazoles 1 (BUB1) Mitotic Checkpoint Serine/Threonine Kinase B (BUB1B), Cyclin A2 (CCNA2), Cyclin B1 (CCNB1), Cyclin B2 (CCNB2), Cell Division Cycle 20 (CDC20), Cyclin-Dependent Kinase (CDK1), Centrosomal Protein of 55 (CEP55), DLG-Associated Protein 5 (DLGAP5), Kinesin Family Member 20A (KIF20A), Kinesin Family Member 2C (KIF2C), Mitotic Arrest Deficient 2 Like 1 (MAD2L1), Nucleolar and Spindle Associated Protein 1 (NUSAP1), Protein Regulator of Cytokinesis 1 (PRC1), Topoisomerase 2-alpha (TOP2A), Targeting protein for Xklp2 (TPX2), Thyroid Hormone Receptor Interactor 13 (TRIP13), Ubiquitin-conjugating Enzyme E2C (UBE2C), and ZW10 Interacting Kinetochore Protein (ZWINT) [Figure 6b]. Later, the heatmap was generated, and their expression patterns were analyzed using the UALCAN web-portal [Figure 7].
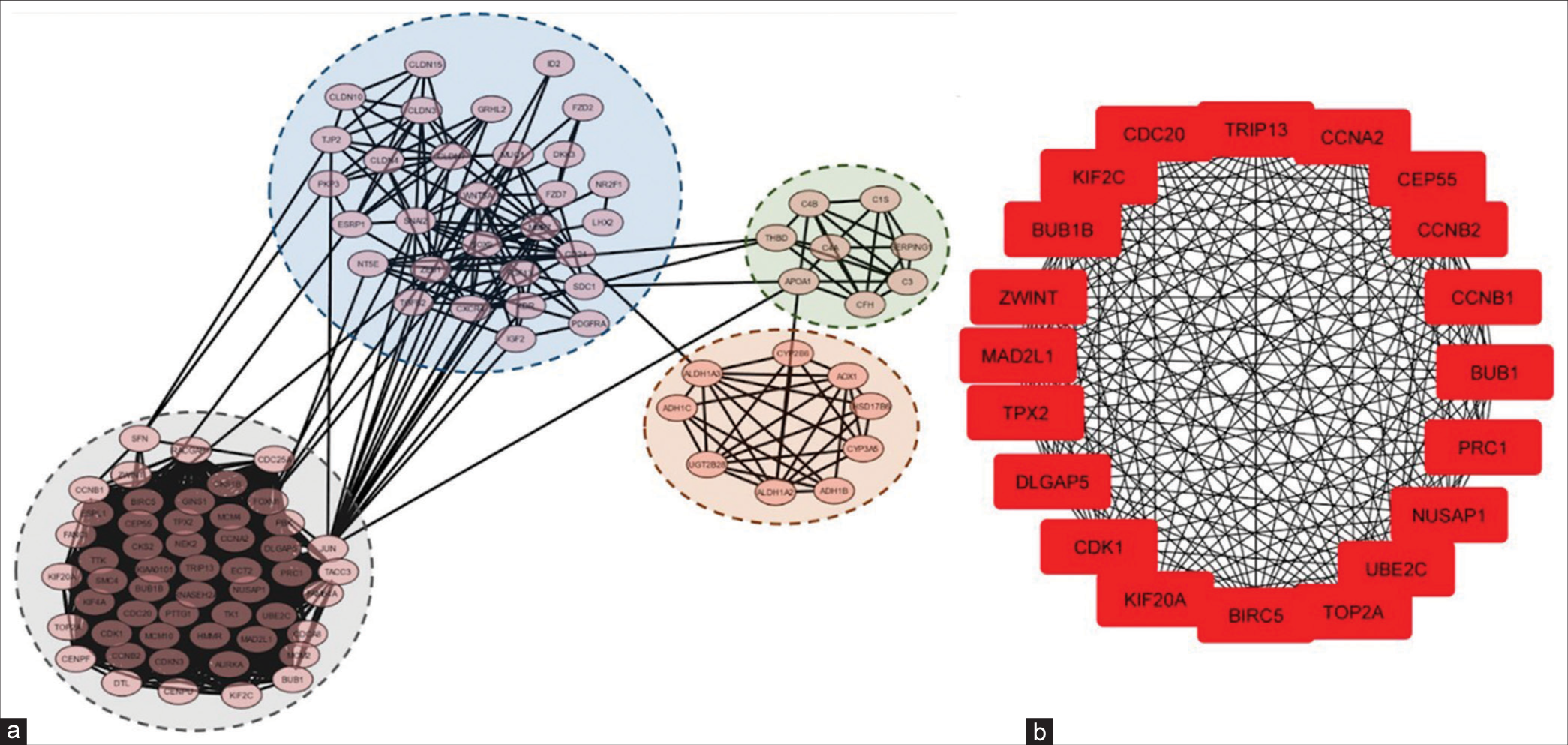
- Module analysis and hub gene identification. (a) The top four modules were screened using MCODE in Cytoscape software. (b) Top twenty hub genes selected by the CytoHubba in Cytoscape based on the degree of each protein node. MCODE: Molecular complex detection.
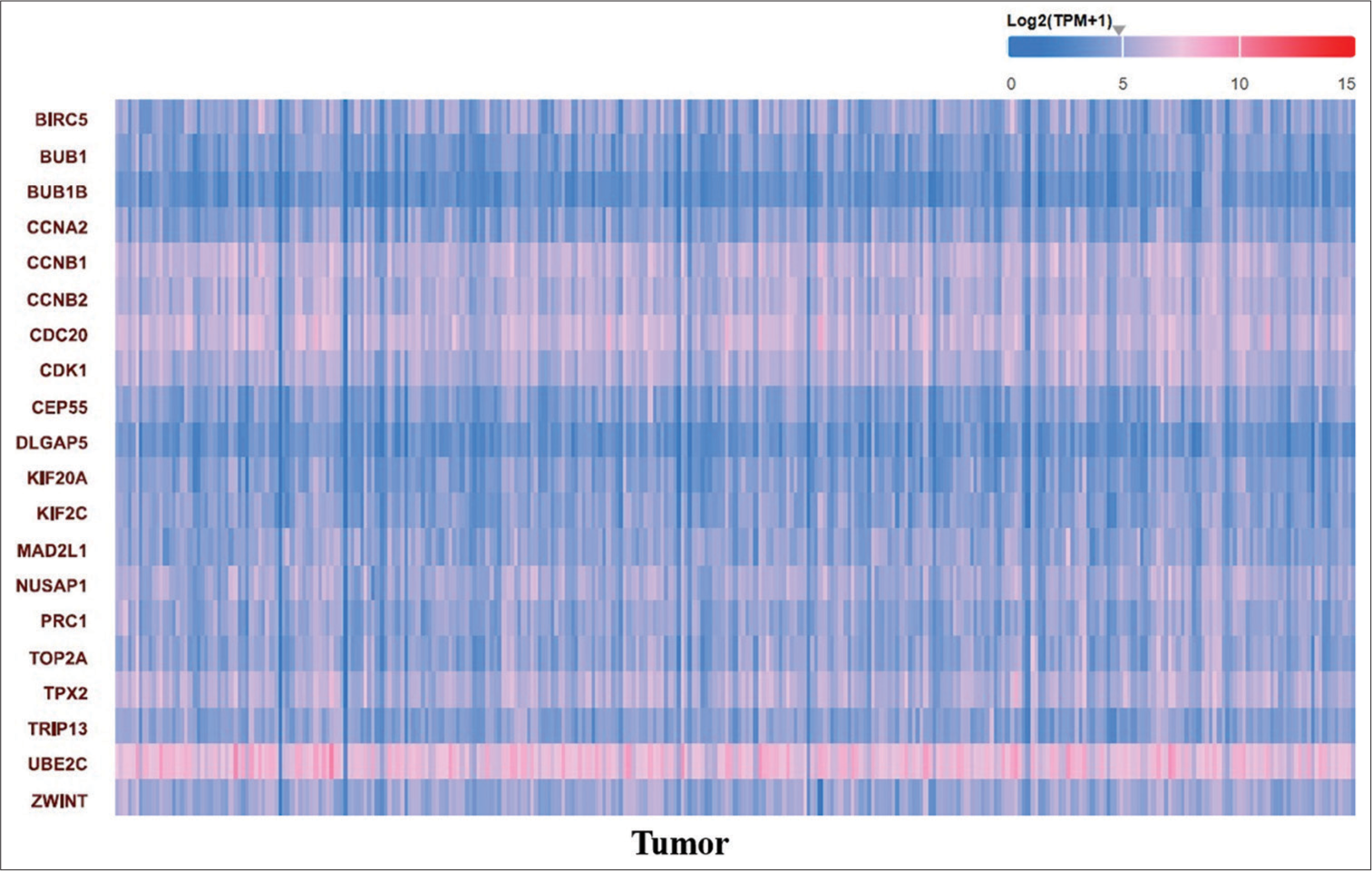
- Heatmap diagram showing the expression pattern of the top 20 candidate hub genes in ovarian adenocarcinoma. The expression level of the gene is represented as log2(TPM+ 1). Red shadow indicates genes over-expressed in tumor samples compared to normal, while green shadow indicates genes under-expressed.
Validation of candidate hub genes
As previously mentioned, the genes identified by module detection algorithms were subjected to survival analysis using the KM plotter, expression pattern analysis, and cancer stage expression analysis. Results from the KM plotter indicated that among the top 20 genes, 17 genes (BUB1, BUB1B, CCNA2, CCNB1, CCNB2, CEP55, DLGAP5, KIF20A, KIF2C, MAD2L1, NUSAP1, PRC1, TOP2A, TPX2, TRIP13, UBE2C, and ZWINT) were associated with survival outcomes in OvCa patients, with higher expression levels correlating with worse overall survival (OS) [Figure 8]. Furthermore, analysis using the GEPIA2 web server revealed that all 17 genes exhibited higher mRNA expression levels in cancerous specimens compared to normal specimens [P < 0.05, Figure 9].
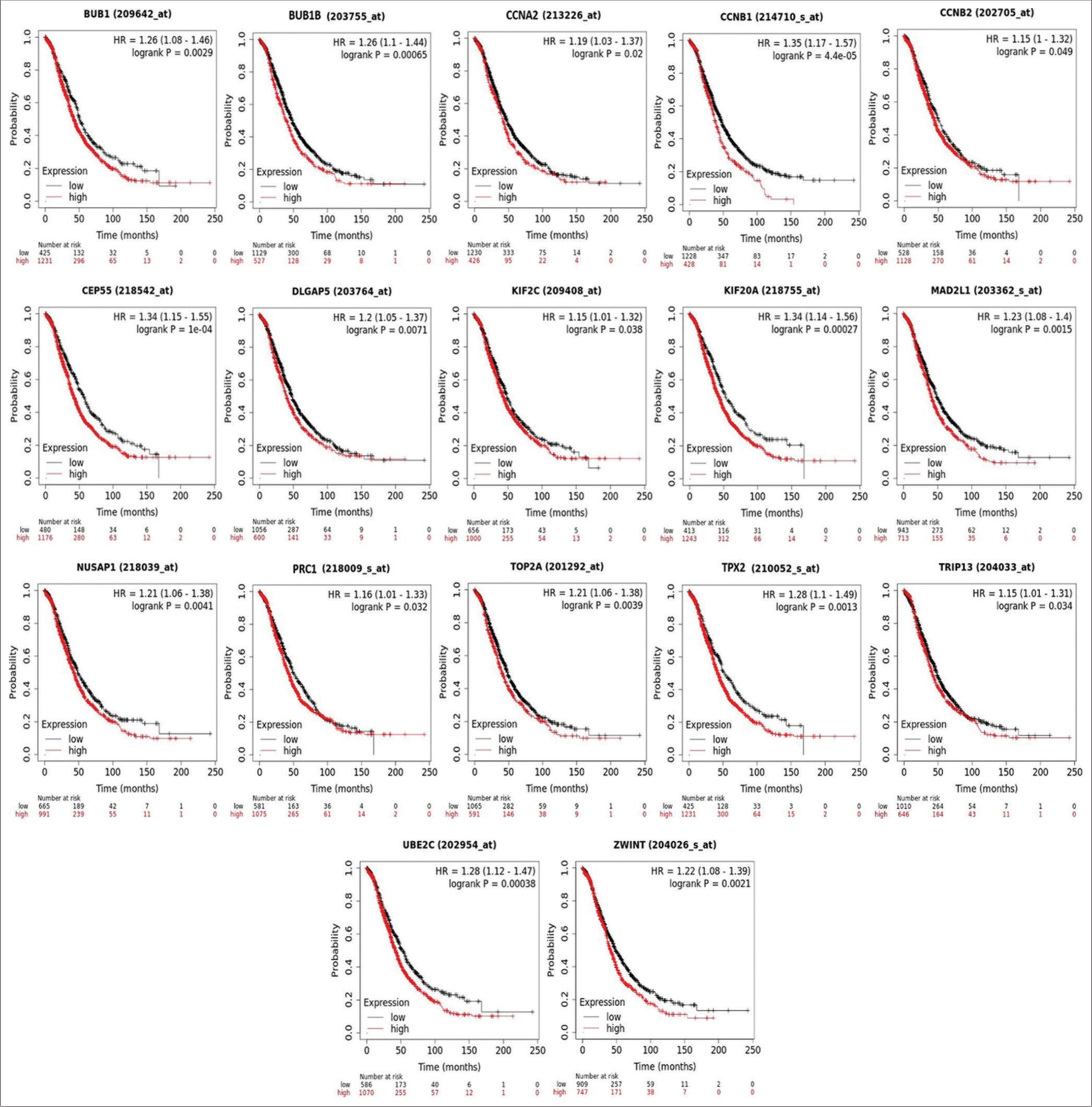
- Kaplan–Meier plots showing the association of identified candidate hub gene expression in the survival of ovarian adenocarcinoma.
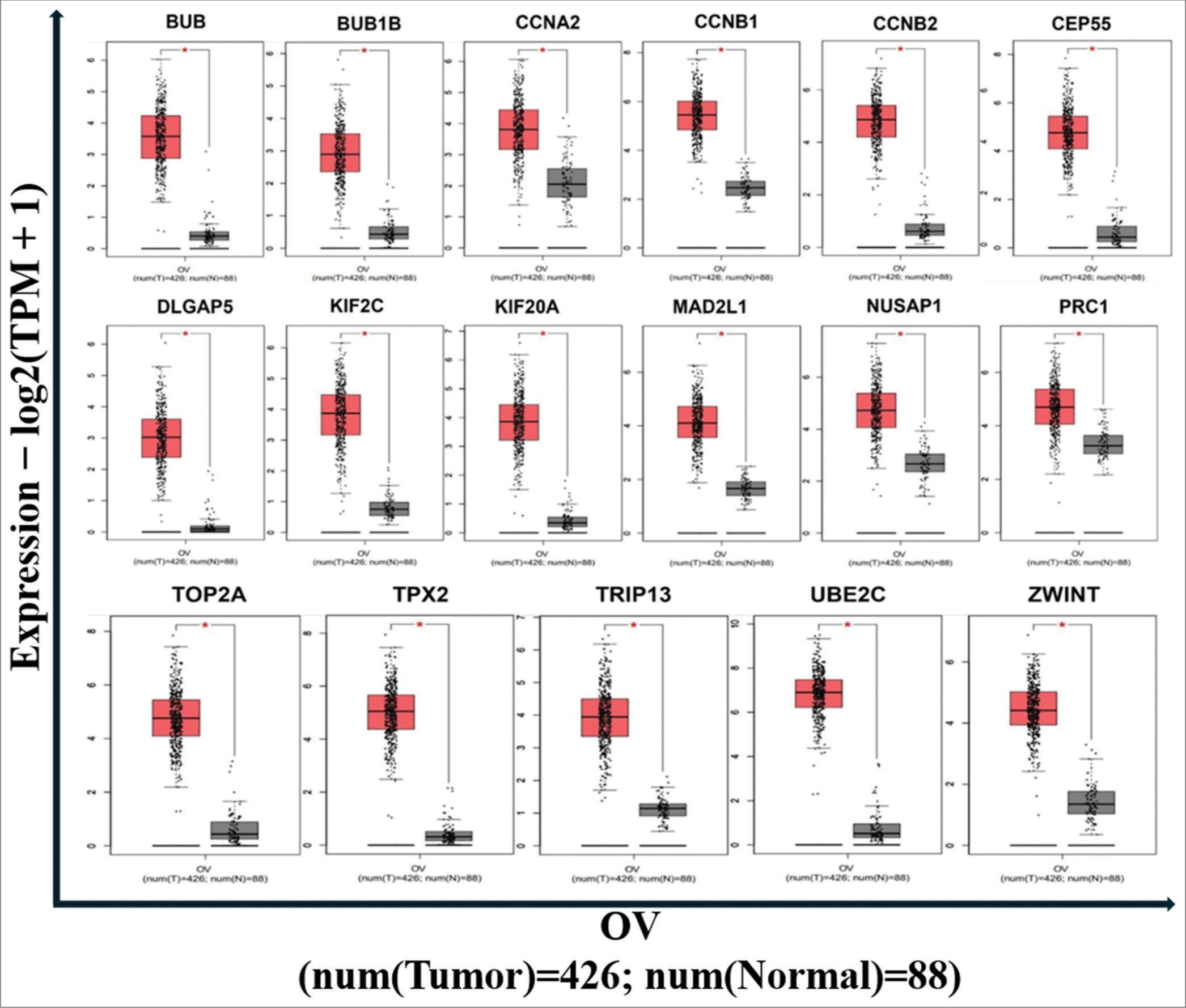
- The expression of hub genes in ovarian cancer samples compared to normal. P < 0.05 was considered significant.
Clinical stage analyses of hub genes
In addition, we investigated the expression levels of the 17 genes across different tumor stages, revealing that only six genes met the inclusive criteria of Pr(>F) < 0.05 [Figure 10]. Notably, significant variations were observed in the expression levels of BUB1B [Pr(>F) = 0.00954], CCNA2 [Pr(>F) = 0.00933], MAD2L1 [Pr(>F) = 0.00446], PRC1 [Pr(>F) = 0.0159], TRIP13 [Pr(>F) = 0.026], and ZWINT [Pr(>F) = 0.0117]. Overall, trends suggested a gradual decrease in the expression of these six genes with the continuous progression of OvCa. However, it is noteworthy that the expression levels remained significantly higher in OvCa tissues compared to normal ovarian tissues, as previously mentioned. The elevated expression levels of CCNA2, MAD2L1, PRC1, and TRIP13 proteins in cancerous samples were further validated by data from the HPA database [Figure 11], although information regarding BUB1B and ZWINT proteins was unavailable in the HPA database. Ultimately, we identified six genes (BUB1B, CCNA2, MAD2L1, PRC1, TRIP13, and ZWINT) as hub genes crucial for OvCa progression.
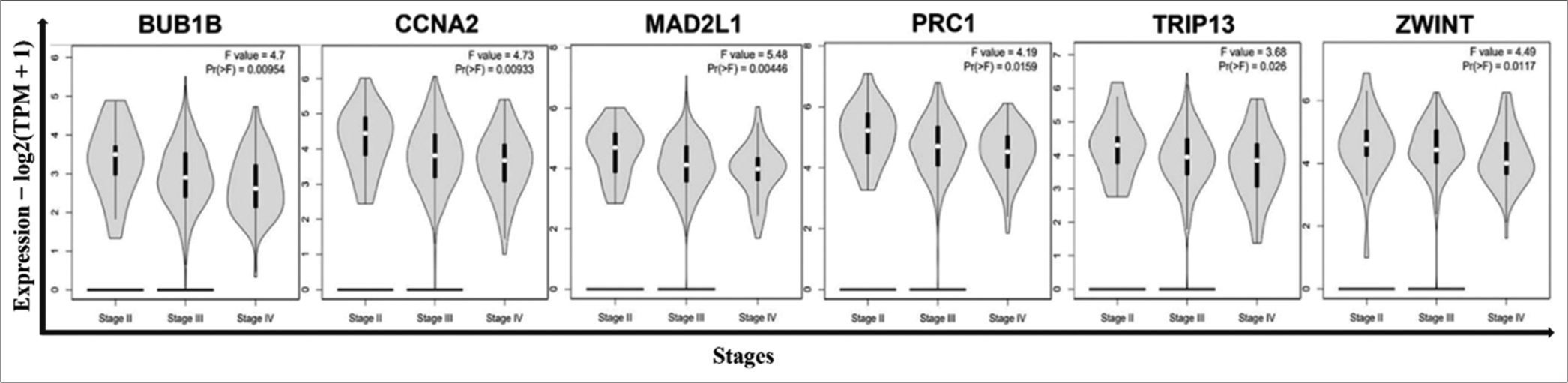
- The expression level of hub genes in OvCa tissues at different stages. To further verify the expression level of the hub genes in OvCa tissues at different stages, the hub genes were analyzed by the GEPIA2 online database. ANOVA was performed to assess the statistical significance of the variations. Pr (>F) < 0.05 was considered statistically significant. According to the result, there were significant variations in the expression levels of BUB1B, CCNA2, MAD2L1, PRC1, TRIP13, and ZWINT. The overall trends indicated that the expression of these six genes decreased gradually with the continuous progression of OvCa. OvCa: Ovarian cancer, GEPIA2: Gene expression profiling interactive analysis 2, ANOVA: Analysis of variance, BUB1B: BUB1 Mitotic Checkpoint Serine/Threonine Kinase B, CCNA2: Cyclin A2, MAD2L1: Mitotic Arrest Deficient 2 Like 1, PRC1: Protein Regulator of Cytokinesis 1, TRIP13: Thyroid Hormone Receptor Interactor 13, ZWINT: ZW10 Interacting Kinetochore Protein.
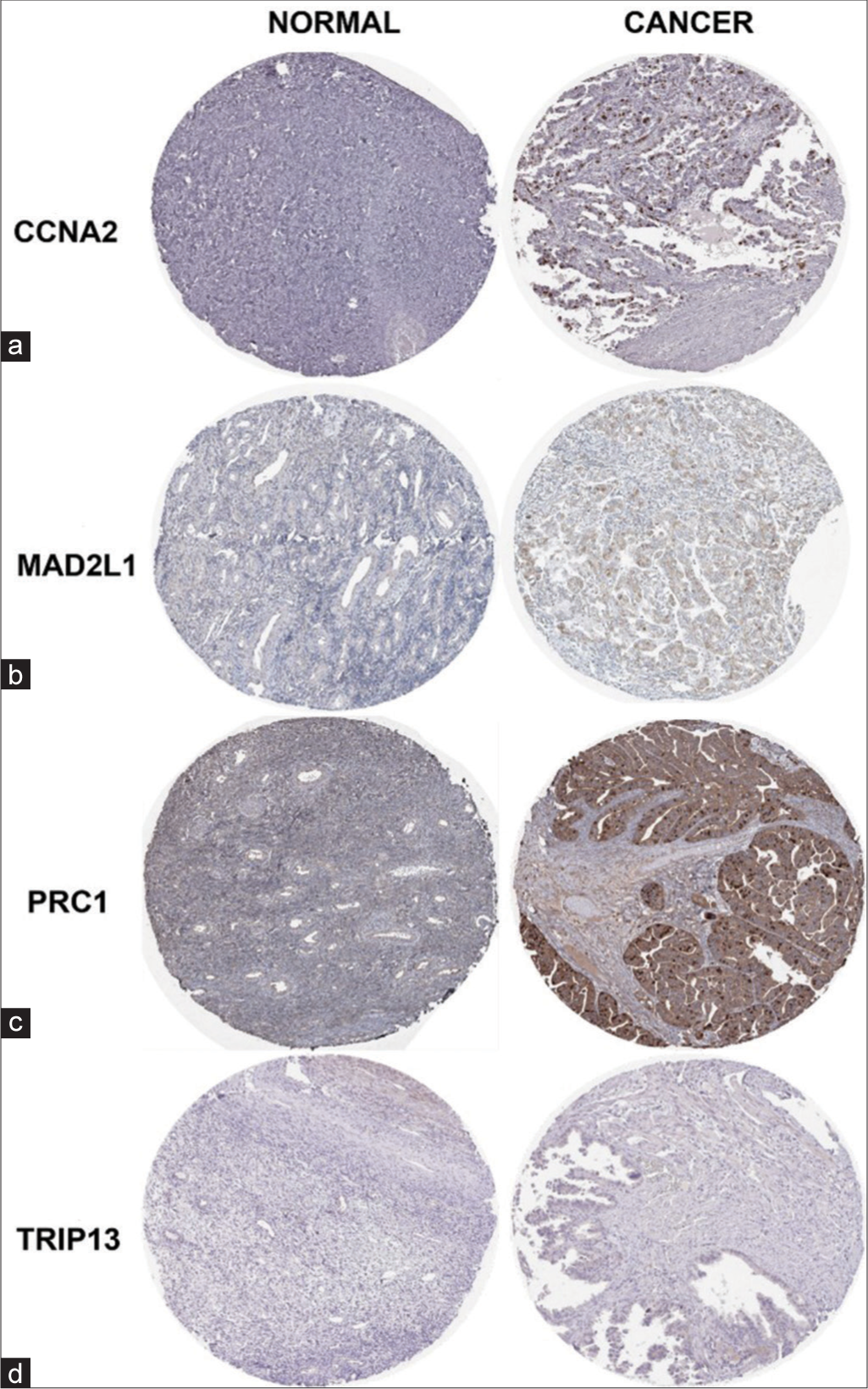
- Validation of hub genes from the HPA database. (a) CCNA2 expression is not detected in the normal samples (antibody CAB000114) however, display high expression levels of CCNA2 (antibody CAB000114) in ovarian cancer tissue. (b) MAD2L1 expression is not detected in the normal samples (antibody HPA003348) and ovarian cancer tissue samples display intermediate expression levels of MAD2L1 (antibody HPA003348). (c) PRC1 expression is not detected in the normal samples (antibody HPA034521) and showed high expression levels of PRC1 (antibody HPA034521) in ovarian cancer tissue samples. (d) TRIP13 expression is not detected in the normal samples (antibody HPA053093). Ovarian cancer samples display high expression levels of TRIP13 (antibody HPA053093). CCNA2: Cyclin A2, MAD2L1: Mitotic Arrest Deficient 2 Like 1, PRC1: Protein Regulator of Cytokinesis 1, TRIP13: Thyroid Hormone Receptor Interactor 13, ZWINT: ZW10 Interacting Kinetochore Protein, HPA: Human protein atlas.
Again, using the STRING database, we constructed the PPI network among the above-mentioned six genes [Figure 12] and we observed that the identified six hub genes are interacting with each other. Later, we also constructed the PPI network of each six identified genes individually, and by this network, we found that (a) each gene was involved in an individual hub gene network, (b) there are certain other proteins interacting with our hub genes (median score- 0.400) [Figure 13, Table 4].
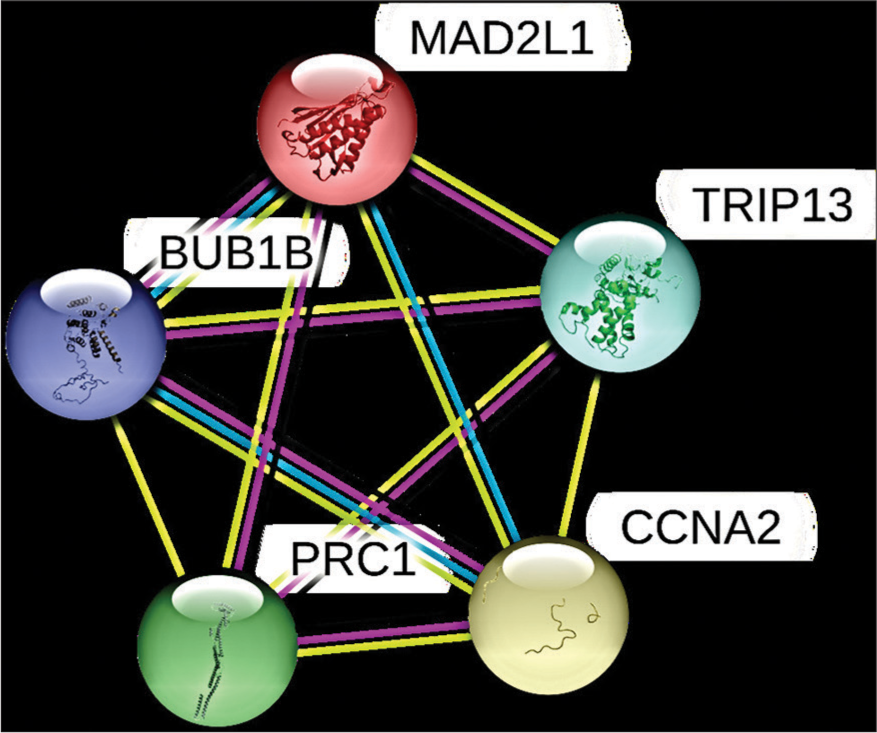
- The PPI network based on STRING database of hub genes (BUB1B, CCNA2, MAD2L1, PRC1, TRIP13, and ZWINT). PPI: Protein-protein interaction, STRING: Search tool for the retrieval of interacting genes, BUB1B: BUB1 Mitotic Checkpoint Serine/Threonine Kinase B, CCNA2: Cyclin A2, MAD2L1: Mitotic Arrest Deficient 2 Like 1, PRC1: Protein Regulator of Cytokinesis 1, TRIP13: Thyroid Hormone Receptor Interactor 13, ZWINT: ZW10 Interacting Kinetochore Protein.
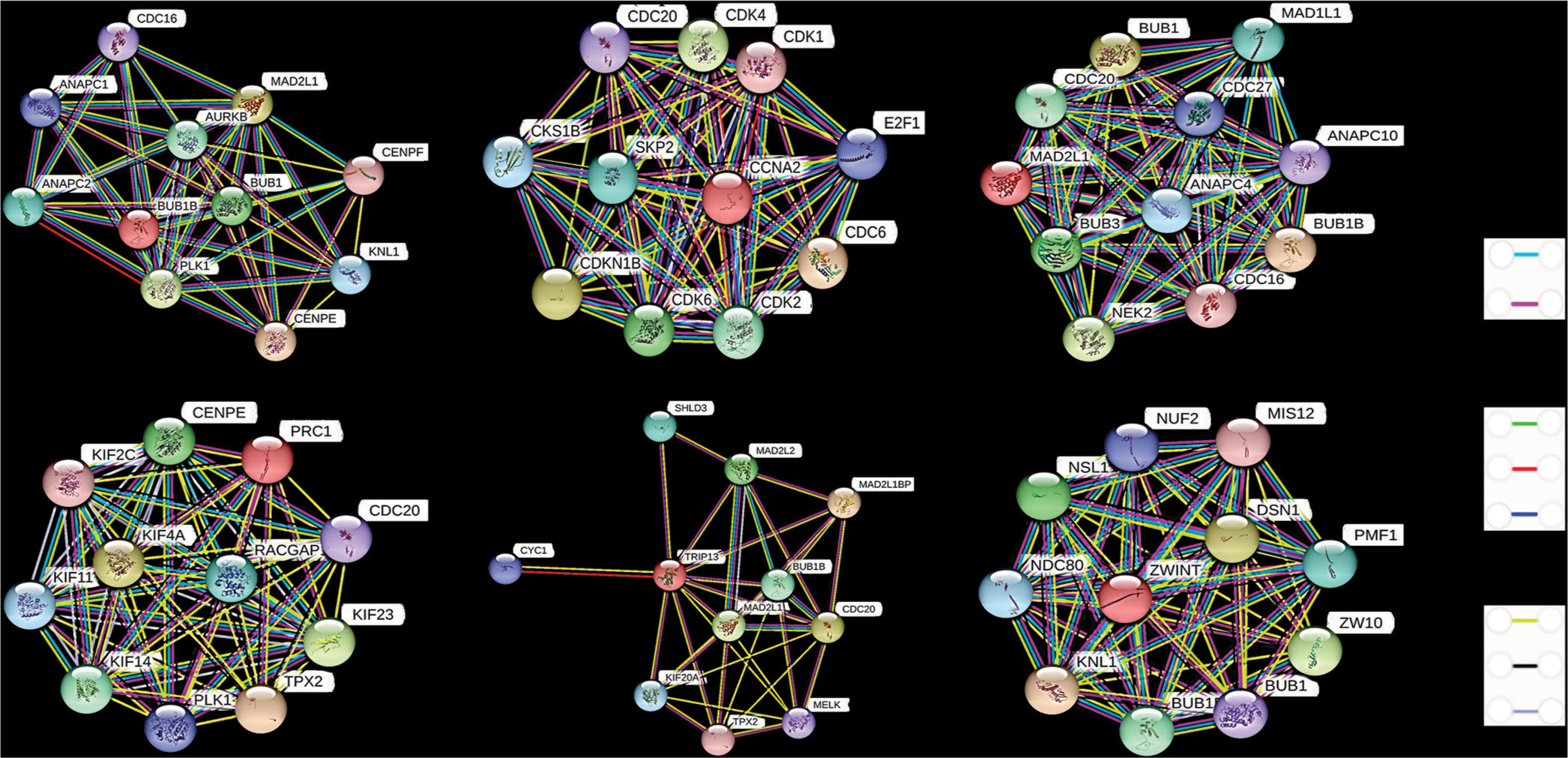
- The PPI network of each hub gene based in STRING database. In the interaction network, genes are denoted by node and their interactions are called edges. The color of the edges is based on their interaction type, i.e., known interactions, predicted interactions, and others. PPI: Protein-protein interaction, STRING: Search tool for the retrieval of interacting genes.
S. No. | Gene | Interacting genes |
---|---|---|
1. | BUB1B | AURKB, ANAPC1, ANAPC2, BUB1, CDC16, CENPE, CENPF, KNL1, MAD2L1, PLK1 |
2. | CCNA2 | CDC20, CDC6, CDK1, CDK2, CDK4, CDK6, CDK1NB, CKS1B, E2F1, SKP2 |
3. | MAD2L1 | ANAPC4, BUB1, BUB1B, BUB3, CDC16, CDC20, CDC27, MAD1L1, NEK2, ANAPC10 |
4. | PRC1 | CENPE, KIF11, KIF14, KIF23, KIF2C, KIF4A, PLK1, RACGAP1, TPX2, CDC20 |
5. | TRIP13 | CDC20, KIF20A, MAD2L1, MAD2L1BP, MAD2L2, MELK, TPX2, TRIP13, BUB1B, KIF20A |
6. | ZWINT | BUB1B, DSN1, KNL1, MIS12, NDN80, NSL1, NUF2, PMF1, ZW10, BUB1 |
PPI: Protein-protein interaction, STRING: Search tool for the retrieval of interacting genes. BUB1B: BUB1 Mitotic Checkpoint Serine/Threonine Kinase B, CCNA2: Cyclin A2, MAD2L1: Mitotic Arrest Deficient 2 Like 1, PRC1: Protein Regulator of Cytokinesis 1, TRIP13: Thyroid Hormone Receptor Interactor 13, ZWINT: ZW10 Interacting Kinetochore Protein
TF-GRN construction
Utilizing NetworkAnalyst, we constructed the TF-GRN comprising the six identified hub genes, which consisted of 98 edges and 80 nodes/TFs [Figure 14]. The path to generate TF-GRN is (a) Upload Data (official gene symbol of hub genes) > (b) Analysis Overview; GRN – TF-gene Interactions (Databases- TRRUST/ENCODE/JASPAR/ChEA) > (c) Network Builder > (d) Network Viewer >(e) Download. To elaborate, BUB1B interacted with 15 TFs; CCNA2 with 14 TFs; MAD2L1 with 15 TFs; PRC1 with 28 TFs; TRIP13 with 15 TFs; and ZWINT with 11 TFs [Table 5]. In addition, several TFs were observed to have interactions with more than one hub gene.
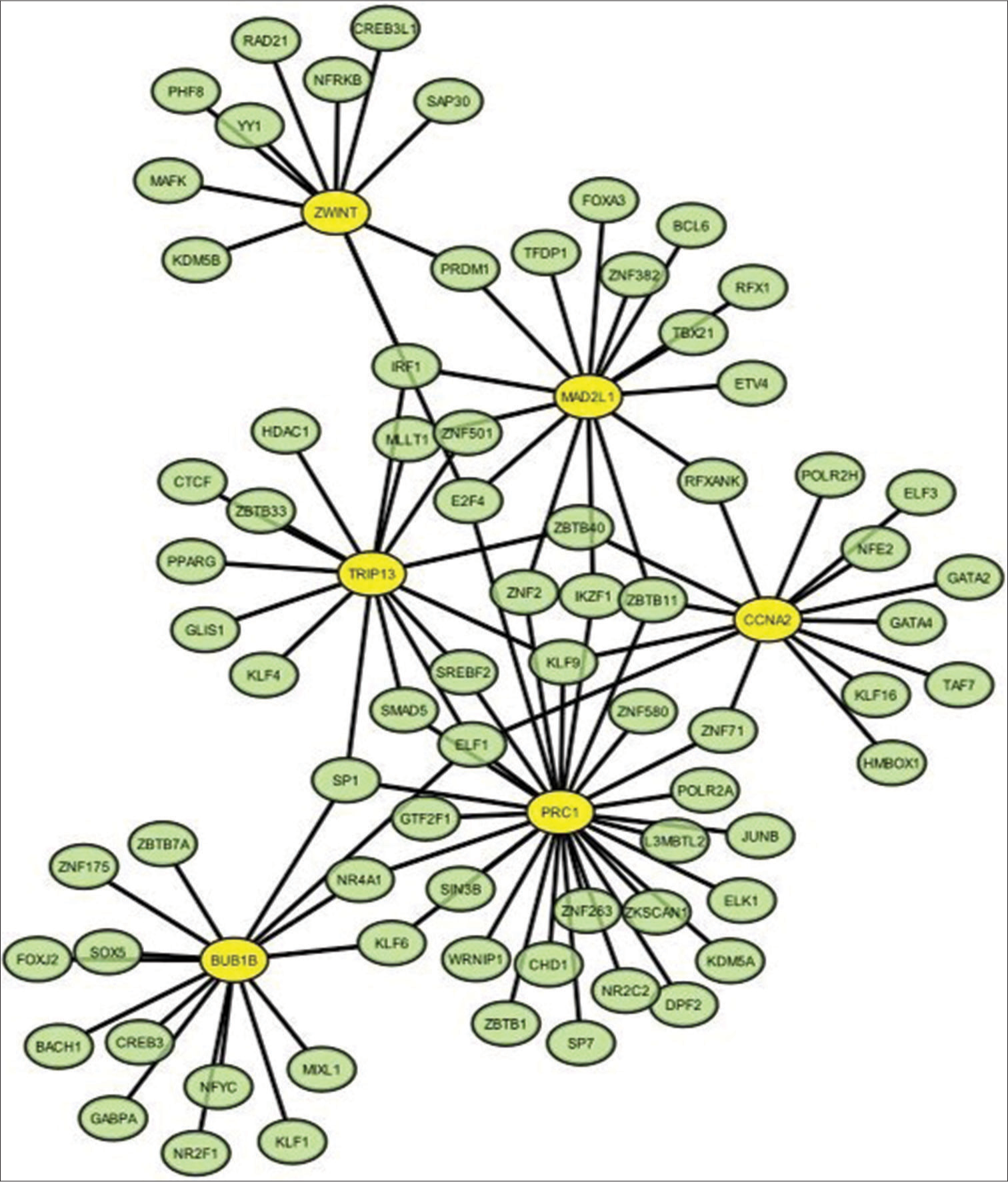
- Transcription factor (TF)-hub gene biomarker regulatory network generated by NetworkAnalyst (version 3.0; http://www.networkanalyst.ca). The network revealed 98 interaction/edges among 6 hub genes and 80 nodes/TFs. The yellow circle stands for the hub gene, and the green circle stands for the TF.
S. No. | Hub gene | Transcription factors |
---|---|---|
1. | BUB1B | ELF1, SP1, ZBTB7A, ZNF175, SOX5, FOXJ2, BACH1, CREB3, GABPA, NR2F1, NFYC, KLF1, MIXL1, KLF6, NR4A1 |
2. | CCNA2 | ELF3, NFE2, GATA2, GATA4, TAF7, KLF16, HMBOX, ZNF71, ELF1, KLF9, ZBTB40, RFXANK, POLR2H, ZBTB11 |
3. | MAD2L1 | E2F4, ZNF2, IKZF1, ZBTB11, RFXANK, ETV4, TBX21, RFX1, ZNF382, BCL6, FOXA3, TFDP1, PRDM1, IRF1, MLLT1 |
4. | PRC1 | GTF2F1, NR4A1, KLF6, SIN3B, WRNIP1, ZBTB1, CHD1, SP7, ZNF263, NR2C2, DPF2, KDM5A, ZKSCAN1, ELK1, L3MBTL2, JUNB, POLR2A, ZNF71, ZNF580, ZBTB11, IKZF1, ZNF2, E2F4, KLF9, SREBF2, ELF1, SMAD5, SP1, |
5. | TRIP13 | ZNF501, HDAC1, IRF1, MLLT1, ZBTB33, KLF4, GLIS1, PPARG, SP1, SMAD5, ELF1, SREBF2, KLF9, ZBTB40, CTCF |
6. | ZWINT | IRF1, KDM5B, MAFK, YY1, PHF8, RAD21, NFRKB, CREB3L1, SAP30, PRDM1, E2F4 |
BUB1B: BUB1 Mitotic Checkpoint Serine/Threonine Kinase B, CCNA2: Cyclin A2, MAD2L1: Mitotic Arrest Deficient 2 Like 1, PRC1: Protein Regulator of Cytokinesis 1, TRIP13: Thyroid Hormone Receptor Interactor 13, ZWINT: ZW10 Interacting Kinetochore Protein
Single-nucleotide variation (SNV), methylation, and pathway activity of hub genes
Confirming their significance in clinical samples, BUB1B, CCNA2, MAD2L1, PRC1, TRIP13, and ZWINT were identified as hub genes and subjected to further analysis in the GSCA database. There is a total of 412 SNVs samples dedicated to OvCa. In our analysis, SNVs were observed in all 13 samples for the six hub genes [Figure 15a]. Notably, PRC1 exhibited the highest SNV frequency among the six hub genes, with 46% observed in the 13 samples. Missense mutation emerged as the predominant mutation type. The methylation pattern of the gene significantly impacted gene expression, as depicted in Figure 15b.
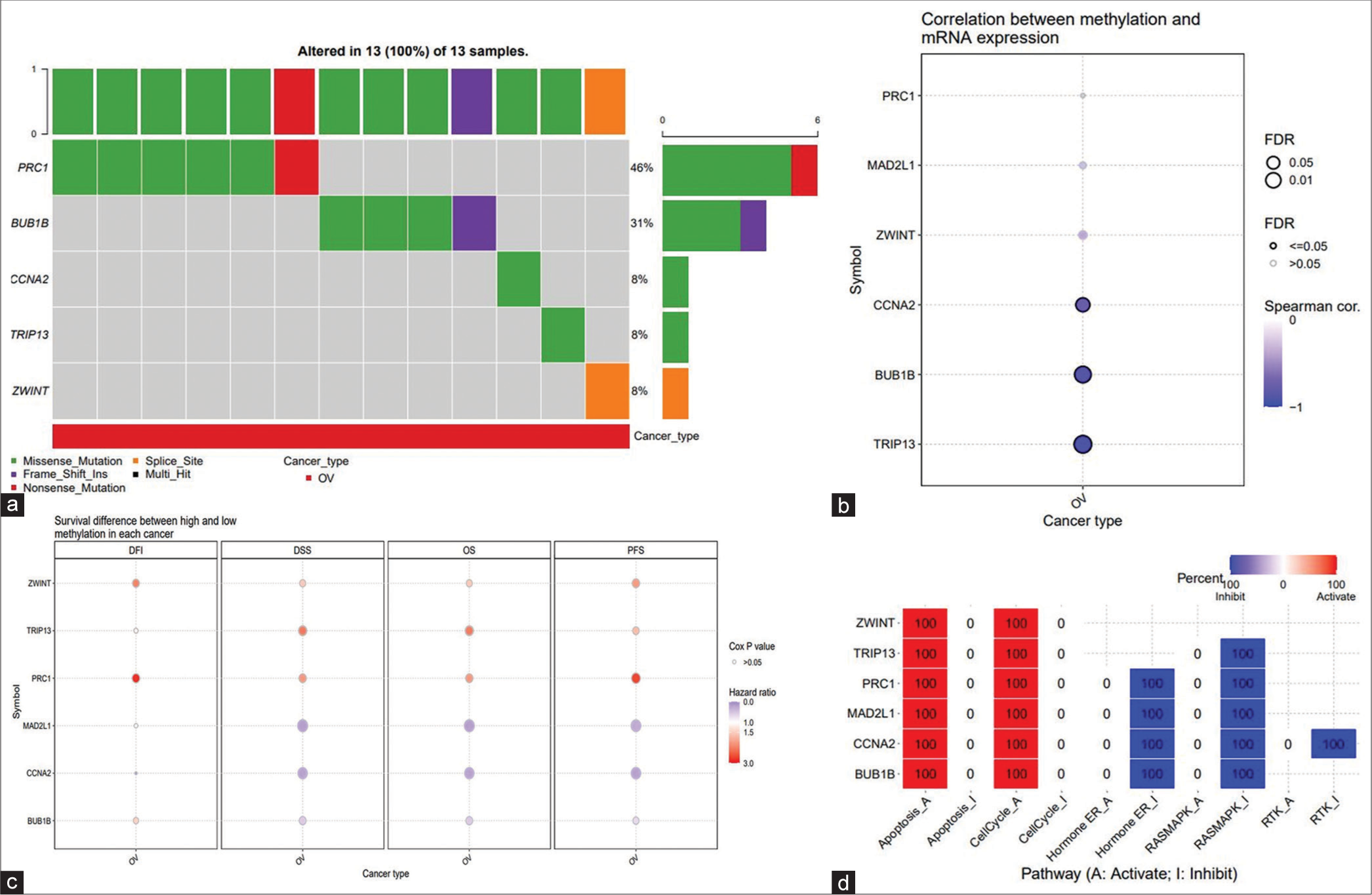
- GSCA online database was appointed to analyze the single-nucleotide variation, methylation, and pathway activity of hub genes. (a) Oncoplot provides a view of the SNV in the OvCa gene set. (b) Correlations between methylation and mRNA expression of hub genes in the OvCa. (c) OS difference between higher and lower methylation groups in the OvCa. (d) Effects of hub gene on cell pathway activity, gene expression was divided into two groups (high and low) by median expression, the difference of PAS between groups was defined by student t-test, FDR adjusted P-value, FDR ≤ 0.05 was considered as significant. The pathway activity module presents the correlation of gene expression with pathway activity groups (activation and inhibition) defined by pathway scores. GSCA: Gene set control analysis, mRNA: Messenger RNA, SNV: Single-nucleotide variation, OS: Overall survival, OvCa: Ovarian cancer, PAS: Pathway activity score.
Further, correlation analysis revealed the association between gene methylation pattern and its effect on mRNA expression [Figure 15c]. In addition, the methylation status of the gene was evaluated concerning OS. Pathway analysis indicated the involvement of all six hub genes in regulating apoptosis and activating the cell cycle. Notably, five hub genes, excluding ZWINT, were implicated in inhibiting the RAS-MAPK pathway [Figure 15d].
Relatively very high level of key gene expression in OvCa cells implicates their role in tumor development and cancer progression
After confirming our findings through bioinformatics analysis, we proceeded to validate the results by conducting RT-PCR to assess the relative mRNA expression levels of BUB1B, CCNA2, MAD2L1, PRC1, TRIP13, and ZWINT in the OvCa cell line, SKOV3, with the human immortalized cell line, HaCaT, serving as the control [Figure 16]. Our experimental results aligned with the previously obtained outcomes, demonstrating elevated mRNA expression levels of BUB1B, CCNA2, MAD2L1, PRC1, TRIP13, and ZWINT in cancer samples compared to normal cells [Primer list provided in Table 6].
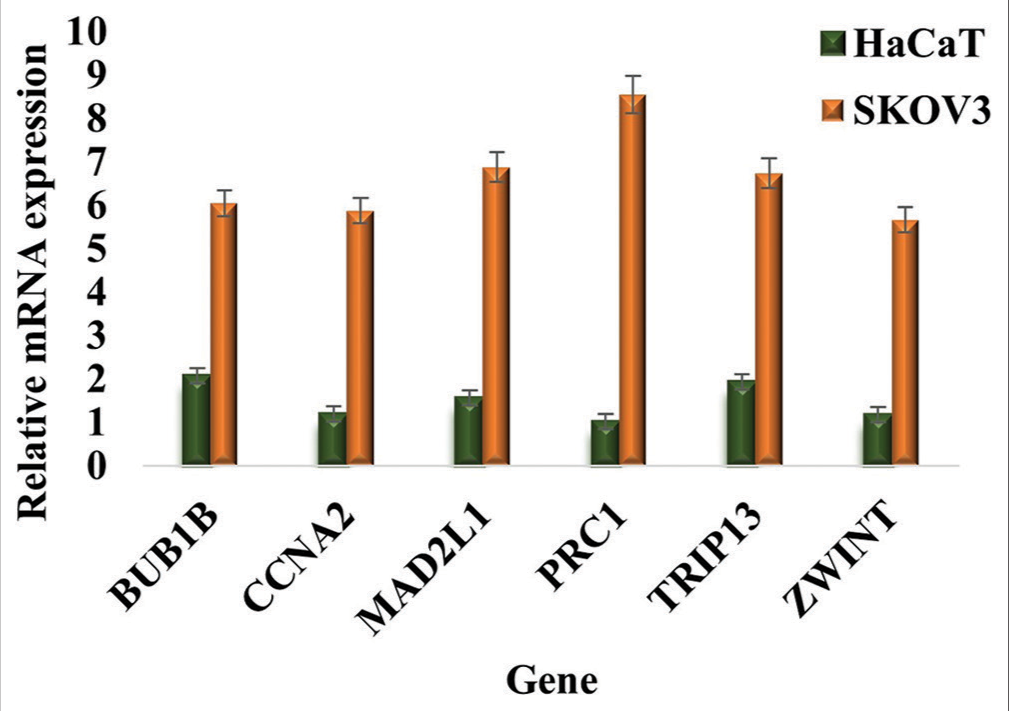
- Expression profiling and relative mRNA expression level of BUB1B, CCNA2, MAD2L1, PRC1, TRIP13, and ZWINT in the ovarian cancer cell line, SKOV3 compared to HaCaT cells (control). The results were normalized to b-Actin and presented as mean ± SD of three independent experiments. mRNA: Messenger RNA, BUB1B: BUB1 Mitotic Checkpoint Serine/Threonine Kinase B, CCNA2: Cyclin A2, MAD2L1: Mitotic Arrest Deficient 2 Like 1, PRC1: Protein Regulator of Cytokinesis 1, TRIP13: Thyroid Hormone Receptor Interactor 13, ZWINT: ZW10 Interacting Kinetochore Protein, SD: Standard deviation.
S. No. | Gene name | Sequence |
---|---|---|
1. | β-Actin | Forward 5'- CTGGAACGGTGAAGGTGACA |
Reverse 5'- AAGGGACTTCCTGTAACAACGCA | ||
2. | BUB1B | Forward 5'-GTGGAAGAGACTGCACAACAGC-3' |
Reverse 5'-TCAGACGCTTGCTGATGGCTCT-3' | ||
3. | CCNA2 | Forward 5'-TTGTAGGCACGGCTGCTATGCT-3' |
Reverse 5'-GGTGCTCCATTCTCAGAACCTG-3' | ||
4. | MAD2L1 | Forward 5'-TTGAGTGTGACAAGACTGCAAAAG-3' |
Reverse 5'-CAGTGGCAGAAATGTCACCGTAG-3' | ||
5. | PRC1 | Forward 5'-ATAGCCAGGAGCAGAGACAAGC-3' |
Reverse 5'-AACCGCACAATCTCAGCATCGTG-3' | ||
6. | TRIP13 | Forward 5'-GTGGACAGCAACCTCATCACCT-3' |
Reverse 5'-TGCTCGACAGTCTGATGGTCAG-3' | ||
7. | ZWINT | Forward 5'-CCTGCCAAGATCATGGAGGAGT-3' |
Reverse 5'-GGCATCTTCAGAAGCCAGAGCA-3' |
qRT-PCR: Quantitative real-time polymerase chain reaction. BUB1B: BUB1 Mitotic Checkpoint Serine/Threonine Kinase B, CCNA2: Cyclin A2, MAD2L1: Mitotic Arrest Deficient 2 Like 1, PRC1: Protein Regulator of Cytokinesis 1, TRIP13: Thyroid Hormone Receptor Interactor 13, ZWINT: ZW10 Interacting Kinetochore Protein
DISCUSSION
Despite efforts to manage OvCa, the prognosis for patients remains dismal.[36] The absence of reliable early diagnostic biomarkers has led to delayed detection, contributing to increased mortality rates among OvCa patients. Consequently, there is an urgent need to delve into the molecular mechanisms underlying tumorigenesis and the progression of OvCa. Identifying efficient early tumor markers could significantly improve patient outcomes.
Advancements in technologies such as microarray, bioinformatics analysis, and sequencing have revolutionized our ability to identify pivotal genetic alterations in cancers, including OvCa. Leveraging bioinformatics tools, we conducted an exhaustive analysis of DEGs from three GEO datasets (GSE18521, GSE26712, and GSE54388), culminating in the identification of 607 common DEGs across these datasets. Further, through functional and pathway enrichment analyses, we discerned significant enrichment of these 607 DEGs in several cancer-related pathways, including pathways in cancer, MAPK signaling pathway, PI3K-Akt signaling pathway, cell cycle, focal adhesion, and Hippo signaling pathway, among others. These findings underscore the potential involvement of these DEGs in the progression of ovarian carcinoma through several carcinogenic signaling pathways. Subsequently, our analysis identified six genes – BUB1B, CCNA2, MAD2L1, PRC1, TRIP13, and ZWINT – as hub genes implicated in OvCa. In Table 7, we have shown these hub genes, their full names, and related functions.
S. No. | Gene Symbol | Full name | Function |
---|---|---|---|
1. | BUB1B | BUB1 Mitotic Checkpoint Serine/Threonine Kinase B | BUB1B is a critical protein in the mitotic checkpoint, ensuring proper chromosome alignment and attachment to spindle microtubules before cell division to prevent aneuploidy. Dysfunction or mutations in BUB1B can lead to chromosomal instability, contributing to cancer development and other congenital conditions. |
2. | CCNA2 | Cyclin A2 | CCNA2 is essential for cell cycle regulation, controlling both the S phase (DNA replication) and the G2/M transition by activating cyclin-dependent kinases (CDKs). Its precise regulation ensures proper cell division, and its dysregulation can contribute to oncogenesis and other cell proliferation disorders. |
3. | MAD2L1 | Mitotic Arrest Deficient 2 Like 1 | MAD2L1 is a key component of the spindle assembly checkpoint, ensuring accurate chromosome segregation by inhibiting the anaphase-promoting complex/cyclosome (APC/C) until all chromosomes are correctly attached to the spindle apparatus. Dysregulation of MAD2L1 can lead to chromosomal instability and is associated with various cancers. |
4. | PRC1 | Protein Regulator of Cytokinesis 1 | PRC1 is crucial for cytokinesis, facilitating the formation of the midzone and the proper separation of daughter cells during cell division by organizing the central spindle microtubules. Dysregulation of PRC1 can disrupt cell division, leading to aneuploidy and contributing to tumorigenesis. |
5. | TRIP13 | Thyroid Hormone Receptor Interactor 13 | TRIP13 is a critical ATPase involved in the spindle assembly checkpoint, promoting the disassembly of checkpoint complexes and facilitating the proper progression of mitosis. Its dysfunction can lead to chromosomal instability and is associated with increased cancer risk. |
6. | ZWINT | ZW10 Interacting Kinetochore Protein | ZWINT is essential for proper kinetochore function during mitosis, facilitating the recruitment of the ZW10 complex to kinetochores and ensuring accurate chromosome alignment and segregation. Its dysregulation can result in chromosomal instability and is linked to cancer progression. |
Survival analyses unveiled a significant correlation between elevated expression levels of these six hub genes and poor OS rates in OvCa patients. Examination of data from the GEPIA2 and HPA database revealed elevated mRNA expression of BUB1B, CCNA2, MAD2L1, PRC1, TRIP13, and ZWINT, alongside increased protein expression of CCNA2, MAD2L1, PRC1, and TRIP13 in OvCa samples, while HPA lacked data for BUB1B and ZWINT. Consequently, this collective evidence identified the set of six genes (BUB1B, CCNA2, MAD2L1, PRC1, TRIP13, and ZWINT) as potential hub genes linked to OvCa.
The BUB1B gene, located on chromosome 15q15, encodes a kinase integral to the spindle checkpoint and shares homology with yeast Mad3.[37] Its involvement in radioresistance and tumor proliferation in glioblastoma has been documented.[38] Inhibition of BUB1B can lead to massive chromosome loss and apoptotic cell death in human cancer cells.[39] However, excessive BUB1B expression has been linked to chromosomal instability across various cancers, including lung, gastric, bladder, and prostate cancers.[40-46] In OvCa, BUB1B has been associated with high grades and serves as an independent prognostic indicator for cancer recurrence. High BUB1B expression correlates with poor OS and high tumor grade, aligning with findings from previous studies. However, the precise role of BUB1B in OvCa tumorigenesis warrants further investigation due to the conflicting evidence.[47]
CCNA2 serves as a crucial regulator of the cell cycle. While it has been identified as a hub gene in other gynecological cancers such as cervical, endometrial, and vulvar cancers, the specific genes associated with it differ from those presented in our report.[48,49] Elevated expression of CCNA2 has been linked to poor survival outcomes in OvCa.[50] Our findings indicate that CCNA2 is overexpressed in OvCa and correlates with prognosis and tumor grade. In addition, another study has reported upregulation of CCNA2 in chemoresistant epithelial OvCa.[51] MAD2L1 functions as a crucial component of the mitotic checkpoint. Its overexpression has been associated with heightened cellular proliferation, migration, and metastasis, ultimately resulting in reduced survival rates across various cancer types.[52-56] PRC1, a member of the microtubule-associated proteins family, plays a crucial role in cytokinesis. Current research highlights its involvement in human carcinogenesis, including breast carcinoma and hepatocellular carcinoma, among others.[57] PRC1 regulates the p21/p27-pRB family molecules and the FAK-paxillin pathway, promoting cell proliferation and cell cycle progression in non-small cell lung cancer.[58] However, elevated PRC1 levels and activity in prostate cancer are associated with epithelial-to-mesenchymal transition and stemness signatures.[59]
TRIP13 is involved in several BPs.[60] Overexpression of TRIP13 has been observed in various tumors, such as head and neck, breast, lung, liver, prostate, gastric, and human chronic lymphoblastic leukemia.[61,62] Silencing TRIP13 has been shown to sensitize tumor cells to chemotherapeutics, indicating its potential as a novel therapeutic target for human cancers. A recent study from Japan suggested that TRIP13 might act as an oncogene in colorectal cancer cells, although the underlying mechanism remains unclear.[63]
The ZW10 interacting kinetochore protein (ZWINT) is primarily enriched in processes related to cell division, including mitotic sister chromatid segregation, kinetochore function, and the mitotic cell cycle checkpoint.[64] It also plays a role in Centromere function and cell growth. Recent studies have reported overexpression of ZWINT in various human malignancies, including prostate, ovarian, bladder, and breast cancers, as well as pulmonary adenocarcinoma and ependymomas.[65-70] Aberrant expression of ZWINT has been associated with chromosome instability signatures and poor prognosis in primary tumors.[71]
Through analysis of public databases such as GEPIA2 and HPA, we further investigated the expression levels of the hub genes in OvCa tissues compared to normal tissues. Our findings revealed that these hub genes exhibit higher expression levels at both the mRNA and protein levels in OvCa tissues. Correlation analysis confirmed strong associations among these genes, consistent with the results obtained from PPI network analysis. These findings suggest that these hub genes may act synergistically in promoting ovarian tumorigenesis.
In another context, during analyses of colon cancer, we found that a set of genes COL1A1, COL1A2, COL4A1, SPP1, SPARC, and THBS2 are the hub genes in colon cancer progression.[72] Tissue patterning largely depends on gene expression, protein functions, and metabolomics.[73] In view of this, it could be hypothesized that there are other factors that control genes’ expression according to tissue and cell preference. From chromatin modifications mediated epigenetic regulation of genes, the mechanism(s) of tissue-specific expression patterns of DEG genes and hub genes could be predicted.[74-78]
CONCLUSION
In this study, we investigated gene expression profiles in OvCa tissue compared to normal tissue using bioinformatics analysis. Our focus was on identifying DEGs that could play crucial roles in the development and metastasis of OvCa. These identified hub genes hold promise as novel potential biomarkers and therapeutic targets for combating OvCa. Here, a total of 607 DEGs where 248 genes were upregulated and 359 downregulated genes and subsequently six key hub genes, BUB1B, CCNA2, MAD2L1, PRC1, TRIP13, and ZWINT, were identified. Gene expression analysis across different molecular subtypes highlighted the significance of the hub genes in more aggressive subtypes of OvCa.
Moreover, BUB1B, CCNA2, MAD2L1, PRC1, TRIP13, and ZWINT likely play crucial roles in the pathogenesis of OvCa. Supporting evidence from existing literature further bolsters the potential involvement of these candidate genes in cancer pathogenesis. Although this study provides strong evidence for future research into the differential expression of genes in OvCa, it is limited by the lack of experimental data on the expression patterns of these hub genes. Nonetheless, the robustness of in silico methods is underscored by the validation through qRT-PCR analysis. mRNA expression analysis suggested that PRC1 expression is comparatively high among other hub genes. Our research offers insights into therapeutic drugs and biomarkers for OvCa, which can be further explored in future studies using clinical samples.
Acknowledgment
Niharika received fellowship from the SERB project No.: EMR/2016/007034 (to S.K.P) and from the institute research scheme. A. Roy is thankful for fellowships under the Institute Research Scheme.
Ethical approval
The research/study is approved by Ethical Committee, National Institute of Technology, Rourkela, Odisha, India certificate reference number is NITR/IEC/2024/M/21, dated 11/03/2024.
Declaration of patient consent
Patient consent not required as patient’s identity is not disclosed or compromised.
Conflicts of interest
There are no conflicts of interest.
Use of artificial intelligence (AI)-assisted technology for manuscript preparation
The authors confirm that there was no use of artificial intelligence (AI)-assisted technology for assisting in the writing or editing of the manuscript and no images were manipulated using AI.
Financial support and sponsorship
Niharika received fellowship from the SERB project No.: EMR/2016/007034 (to SKP) and from the institute research scheme. A. Roy is thankful for fellowships under the Institute Research Scheme.
References
- Global cancer statistics 2018: GLOBOCAN estimates of incidence and mortality worldwide for 36 cancers in 185 countries. CA Cancer J Clin. 2018;68:394-424.
- [CrossRef] [PubMed] [Google Scholar]
- Cancer treatment and survivorship statistics, 2019. CA Cancer J Clin. 2019;69:363-85.
- [CrossRef] [PubMed] [Google Scholar]
- Minireview: Human ovarian cancer: Biology, current management, and paths to personalizing therapy. Endocrinology. 2012;153:1593-602.
- [CrossRef] [PubMed] [Google Scholar]
- Phytochemicals from Ayurvedic plants as potential medicaments for ovarian cancer: An in silico analysis. J Mol Model. 2021;27:114.
- [CrossRef] [PubMed] [Google Scholar]
- Building a lung and ovarian cancer data warehouse. Healthc Inform Res. 2020;26:303-10.
- [CrossRef] [PubMed] [Google Scholar]
- Ovarian cancer overview: Molecular biology and its potential clinical application. London: Intechopen; 2018:57-82.
- [CrossRef] [Google Scholar]
- Gene expression profiling supports the hypothesis that human ovarian surface epithelia are multipotent and capable of serving as ovarian cancer initiating cells. BMC Med Genomics. 2009;2:71.
- [CrossRef] [PubMed] [Google Scholar]
- Screening based on the risk of cancer calculation from Bayesian hierarchical change point and mixture models of longitudinal markers. J Am Stat Assoc. 2001;96:429-39.
- [CrossRef] [Google Scholar]
- Current clinical application of serum biomarkers to detect ovarian cancer. Prz Menopauzalny. 2015;14:254-9.
- [CrossRef] [PubMed] [Google Scholar]
- A review of Doppler ultrasound quality assurance protocols and test devices. Phys Med. 2014;30:742-51.
- [CrossRef] [PubMed] [Google Scholar]
- Image-guided biopsy: What the interventional radiologist needs to know about PET/CT. Radiographics. 2012;32:1483-501.
- [CrossRef] [PubMed] [Google Scholar]
- Screening for early familial ovarian cancer with transvaginal ultrasonography and colour blood flow imaging. BMJ. 1993;306:1025-9.
- [CrossRef] [PubMed] [Google Scholar]
- Bioinformatics analysis revealing prognostic significance of RRM2 gene in breast cancer. Bioscience reports. 2019;39:BSR20182062.
- [CrossRef] [PubMed] [Google Scholar]
- Integrated bioinformatics analysis for the screening of hub genes and therapeutic drugs in ovarian cancer. J Ovarian Res. 2020;13:10.
- [CrossRef] [PubMed] [Google Scholar]
- Gene expression omnibus: NCBI gene expression and hybridization array data repository. Nucleic Acids Res. 2002;30:207-10.
- [CrossRef] [PubMed] [Google Scholar]
- The gene expression omnibus database. Methods Mol Biol. 2016;1418:93-110.
- [CrossRef] [PubMed] [Google Scholar]
- A gene signature predictive for outcome in advanced ovarian cancer identifies a survival factor: Microfibril-associated glycoprotein 2. Cancer Cell. 2009;16:521-32.
- [CrossRef] [PubMed] [Google Scholar]
- A gene signature predicting for survival in suboptimally debulked patients with ovarian cancer. Cancer Res. 2008;68:5478-86.
- [CrossRef] [PubMed] [Google Scholar]
- Creation of a human secretome: A novel composite library of human secreted proteins: Validation using ovarian cancer gene expression data and a virtual secretome array. Clin Cancer Res. 2015;21:4960-9.
- [CrossRef] [PubMed] [Google Scholar]
- ELF3 is a negative regulator of epithelial-mesenchymal transition in ovarian cancer cells. Oncotarget. 2017;8:16951-63.
- [CrossRef] [PubMed] [Google Scholar]
- DAVID: A web server for functional enrichment analysis and functional annotation of gene lists (2021 update) Nucleic Acids Res. 2022;50:W216-21.
- [CrossRef] [PubMed] [Google Scholar]
- Protocol update for large-scale genome and gene function analysis with the PANTHER classification system (v.14.0) Nat Protoc. 2019;14:703-21.
- [CrossRef] [PubMed] [Google Scholar]
- GeneWeaver: Finding consilience in heterogeneous cross-species functional genomics data. Mamm Genome. 2015;26:556-66.
- [CrossRef] [PubMed] [Google Scholar]
- STRING: A database of predicted functional associations between proteins. Nucleic Acids Res. 2003;31:258-61.
- [CrossRef] [PubMed] [Google Scholar]
- Cytoscape: A software environment for integrated models of biomolecular interaction networks. Genome Res. 2003;13:2498-504.
- [CrossRef] [PubMed] [Google Scholar]
- An automated method for finding molecular complexes in large protein interaction networks. BMC Bioinform. 2003;4:2.
- [CrossRef] [PubMed] [Google Scholar]
- Computing topological parameters of biological networks. Bioinformatics (Oxford, England). 2008;24:282-4.
- [CrossRef] [PubMed] [Google Scholar]
- cytoHubba: Identifying hub objects and sub-networks from complex interactome. BMC Syst Biol. 2014;8(Suppl 4):S11.
- [CrossRef] [PubMed] [Google Scholar]
- GEPIA2: An enhanced web server for large-scale expression profiling and interactive analysis. Nucleic Acids Res. 2019;47:W556-60.
- [CrossRef] [PubMed] [Google Scholar]
- The genotype-tissue expression (GTEx) project. Nat Genet. 2013;45:580-5.
- [CrossRef] [PubMed] [Google Scholar]
- The human protein atlas: A spatial map of the human proteome. Protein Sci. 2018;27:233-44.
- [CrossRef] [PubMed] [Google Scholar]
- NetworkAnalyst 3.0: A visual analytics platform for comprehensive gene expression profiling and meta-analysis. Nucleic Acids Res. 2019;47:W234-41.
- [CrossRef] [PubMed] [Google Scholar]
- NetworkAnalyst--integrative approaches for protein-protein interaction network analysis and visual exploration. Nucleic Acids Res. 2014;42:W167-74.
- [CrossRef] [PubMed] [Google Scholar]
- Ovarian cancer In: Study guide StatPearls. Treasure Island, FL: StatPearls Publishing; 2023.
- [Google Scholar]
- Identification of FoxM1/Bub1b signaling pathway as a required component for growth and survival of rhabdomyosarcoma. Cancer Res. 2012;72:5889-99.
- [CrossRef] [PubMed] [Google Scholar]
- The FOXM1/BUB1B signaling pathway is essential for the tumorigenicity and radioresistance of glioblastoma. Oncol Rep. 2017;38:3367-75.
- [CrossRef] [PubMed] [Google Scholar]
- Lethality to human cancer cells through massive chromosome loss by inhibition of the mitotic checkpoint. Proc Natl Acad Sci U S A. 2004;101:8699-704.
- [CrossRef] [PubMed] [Google Scholar]
- Genomic instability and increased expression of BUB1B and MAD2L1 genes in ductal breast carcinoma. Cancer Lett. 2007;254:298-307.
- [CrossRef] [PubMed] [Google Scholar]
- Overexpression of BUBR1 is associated with chromosomal instability in bladder cancer. Cancer Genet Cytogenet. 2007;174:42-7.
- [CrossRef] [PubMed] [Google Scholar]
- Overexpression of the mitotic checkpoint genes BUB1 and BUBR1 is associated with genomic complexity in clear cell kidney carcinomas. Cell Oncol. 2008;30:389-95.
- [CrossRef] [PubMed] [Google Scholar]
- Requirement for BUB1B/BUBR1 in tumor progression of lung adenocarcinoma. Genes Cancer. 2015;6:106-18.
- [CrossRef] [PubMed] [Google Scholar]
- BUBR1 insufficiency is correlated with eNOS reduction experimentally in vitro and in vivo, and in gastric cancer tissue. Anticancer Res. 2018;38:6099-106.
- [CrossRef] [PubMed] [Google Scholar]
- BUBR1 overexpression predicts disease-specific survival after nephroureterectomy in patients with upper tract urothelial carcinoma. Jpn J Clin Oncol. 2016;46:754-61.
- [CrossRef] [PubMed] [Google Scholar]
- Overexpression of BUB1B, CCNA2, CDC20, and CDK1 in tumor tissues predicts poor survival in pancreatic ductal adenocarcinoma. Biosci Rep. 2019;39:BSR20182306.
- [CrossRef] [PubMed] [Google Scholar]
- BubR1 as a prognostic marker for recurrence-free survival rates in epithelial ovarian cancers. Br J Cancer. 2009;101:504-10.
- [CrossRef] [PubMed] [Google Scholar]
- Bioinformatics prediction and analysis of hub genes and pathways of three types of gynecological cancer. Oncol Lett. 2019;18:617-28.
- [CrossRef] [PubMed] [Google Scholar]
- Identification of potential crucial genes associated with the pathogenesis and prognosis of endometrial cancer. Front Genet. 2019;10:373.
- [CrossRef] [PubMed] [Google Scholar]
- miR-508-3p suppresses the development of ovarian carcinoma by targeting CCNA2 and MMP7. Int J Oncol. 2020;57:264-76.
- [CrossRef] [PubMed] [Google Scholar]
- Identification of genes with differential expression in chemoresistant epithelial ovarian cancer using high-density oligonucleotide microarrays. Oncol Res. 2009;18:47-56.
- [CrossRef] [PubMed] [Google Scholar]
- High expression of spindle assembly checkpoint proteins CDC20 and MAD2 is associated with poor prognosis in urothelial bladder cancer. Virch Arch. 2013;463:681-7.
- [CrossRef] [PubMed] [Google Scholar]
- Spindle proteins in resected pancreatic head adenocarcinomas: BubR1 is an independent prognostic factor in pancreatobiliary-type tumours. Histopathology. 2010;56:345-55.
- [CrossRef] [PubMed] [Google Scholar]
- Proteins of the mitotic checkpoint and spindle are related to chromosomal instability and unfavourable prognosis in patients with myelodysplastic syndrome. J Clin Pathol. 2015;68:381-7.
- [CrossRef] [PubMed] [Google Scholar]
- Combination analysis of Bub1 and Mad2 expression in endometrial cancer: Act as a prognostic factor in endometrial cancer. Arch Gynecol Obstet. 2013;288:155-65.
- [CrossRef] [PubMed] [Google Scholar]
- Mad2 checkpoint gene silencing using epidermal growth factor receptor-targeted chitosan nanoparticles in non-small cell lung cancer model. Mol Pharm. 2014;11:3515-27.
- [CrossRef] [PubMed] [Google Scholar]
- PRC1 promotes cell proliferation and cell cycle progression by regulating p21/p27-pRB family molecules and FAK-paxillin pathway in non-small cell lung cancer. Translat Cancer Res. 2019;8:2059-72.
- [CrossRef] [PubMed] [Google Scholar]
- PRC1 contributes to tumorigenesis of lung adenocarcinoma in association with the Wnt/β-catenin signaling pathway. Mol Cancer. 2017;16:108.
- [CrossRef] [PubMed] [Google Scholar]
- Mechanisms of Polycomb group protein function in cancer. Cell Res. 2022;32:231-53.
- [CrossRef] [PubMed] [Google Scholar]
- Insights into a crucial role of TRIP13 in human cancer. Comput Struct Biotechnol J. 2019;17:854-61.
- [CrossRef] [PubMed] [Google Scholar]
- Thyroid receptor-interacting protein 13 is correlated with progression and poor prognosis in bladder cancer. Med Sci Monit. 2019;25:6660-8.
- [CrossRef] [PubMed] [Google Scholar]
- Biallelic TRIP13 mutations predispose to Wilms tumor and chromosome missegregation. Nat Genet. 2017;49:1148-51.
- [CrossRef] [PubMed] [Google Scholar]
- TRIP13 promotes metastasis of colorectal cancer regardless of p53 and microsatellite instability status. Mol Oncol. 2020;14:3007-29.
- [CrossRef] [PubMed] [Google Scholar]
- HZwint-1, a novel human kinetochore component that interacts with HZW10. J Cell Sci. 2000;113:1939-50.
- [CrossRef] [PubMed] [Google Scholar]
- A census of mitotic cancer genes: New insights into tumor cell biology and cancer therapy. Carcinogenesis. 2007;28:899-912.
- [CrossRef] [PubMed] [Google Scholar]
- Classification of human lung carcinomas by mRNA expression profiling reveals distinct adenocarcinoma subclasses. Proc Natl Acad Sci U S A. 2001;98:13790-5.
- [CrossRef] [PubMed] [Google Scholar]
- Prognostic model of pulmonary adenocarcinoma by expression profiling of eight genes as determined by quantitative real-time reverse transcriptase polymerase chain reaction. J Clin Oncol. 2004;22:811-9.
- [CrossRef] [PubMed] [Google Scholar]
- Deregulation of Rab and Rab effector genes in bladder cancer. PLoS One. 2012;7:e39469.
- [CrossRef] [PubMed] [Google Scholar]
- Overexpression of androgen receptor enhances the binding of the receptor to the chromatin in prostate cancer. Oncogene. 2012;31:2153-63.
- [CrossRef] [PubMed] [Google Scholar]
- Identification of candidate biomarkers and analysis of prognostic values in ovarian cancer by integrated bioinformatics analysis. Med Oncol. 2016;33:130.
- [CrossRef] [PubMed] [Google Scholar]
- Genomics and epigenetics: A study of ependymomas in pediatric patients. Clin Neurol Neurosurg. 2016;144:53-8.
- [CrossRef] [PubMed] [Google Scholar]
- Deciphering the link between membrane signaling and hub genes: Bioinformatics prediction and experimental validation in colon cancer. Research Square [Preprint] 2022
- [CrossRef] [Google Scholar]
- The effect of tissue composition on gene co-expression. Brief Bioinform. 2021;22:127-39.
- [CrossRef] [PubMed] [Google Scholar]
- KDM5A noncanonically binds antagonists MLL1/2 to mediate gene regulation and promotes EMT. Biochim Biophys Acta Gene Regul Mech. 2023;1866:194986.
- [CrossRef] [PubMed] [Google Scholar]
- Epigenetic signaling and crosstalk in regulation of gene expression and disease progression. Epigenomics. 2023;15:723-40.
- [CrossRef] [PubMed] [Google Scholar]
- Overexpression of SOX2 gene by histone modifications: SOX2 enhances human prostate and breast cancer progression by prevention of apoptosis and enhancing cell proliferation. Oncology. 2023;101:591-608.
- [CrossRef] [PubMed] [Google Scholar]
- Epigenetic regulation of pluripotency inducer genes NANOG and SOX2 in human prostate cancer. Prog Mol Biol Transl Sci. 2023;197:241-60.
- [CrossRef] [PubMed] [Google Scholar]
- Dissecting SOX2 expression and function reveals an association with multiple signaling pathways during embryonic development and in cancer progression. Biochimica Biophys Acta Rev Cancer. 2024;1879:189136.
- [CrossRef] [PubMed] [Google Scholar]